Introduction
Why is everyone talking about generative AI?
Generative AI has been around for several decades, so why all the buzz right now? The short answer is that new innovations like Large Language Models (LLMs) have made AI approachable to the masses with a simple, user-friendly interface and versatility across diverse use cases.
ChatGPT, arguably the fastest growing app of all times, had over a million users 5 days after its launch and has continued its incredible trajectory to over 180 million users just one year later. Its dominance is well-earned, considering the value it’s driven across a wide range of use cases, such as content development, research, translation, and beyond.
Enterprises are taking notice. According to a recent survey by McKinsey, three-quarters of participants anticipate that generative AI will bring about significant or disruptive changes in their industry's competitive landscape within the next three years.
LLMs hold immense potential for enterprises, however it will take some time to identify their strategic application beyond chatbot functionalities and operationalize them on a larger scale. While this exploration unfolds, a novel generative AI technology is emerging: Large Graphical Models (LGMs). In this eBook, we’ll take a look at the evolution of generative AI to get a better understanding of the various models and their capabilities, limitations, and areas of opportunity.
What is Generative AI?
An overview of generative AI
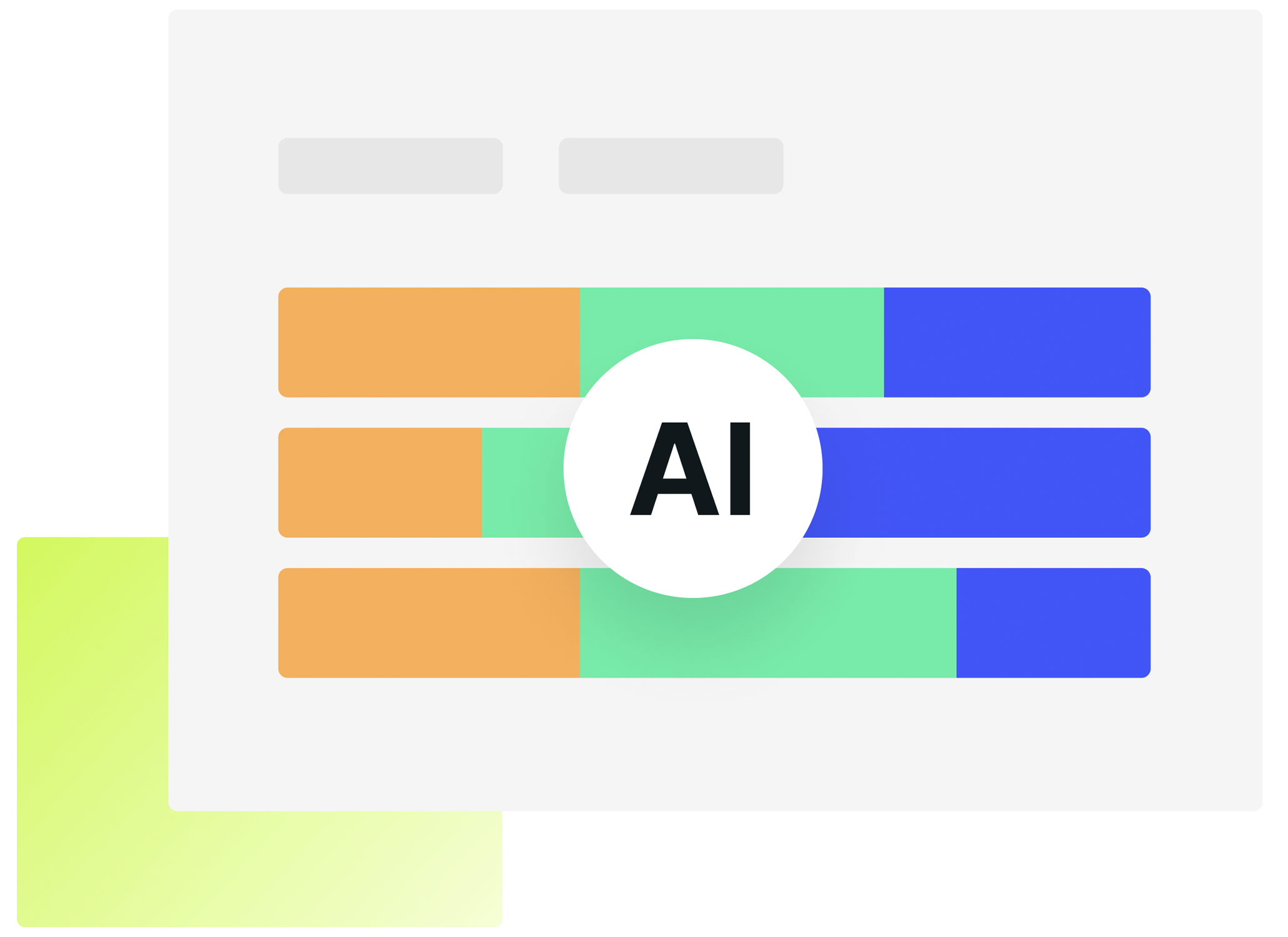
Generative AI is a category of artificial intelligence that ingests and learns from massive datasets with the goal of creating new content, such as text, audio, and images. It works by learning the underlying structure, finding patterns in existing data to generate new content.
While we are still in the early – and mostly experimental - days of AI adoption in the enterprise, there is no doubt that business leaders see its strategic value and are racing to capitalize on its potential. In fact, McKinsey Global Institute estimates that generative AI will add between $2.6 and $4.4 trillion in annual value to the global economy, increasing the economic impact of AI by 15 to 40%. The most notable example of generative AI in natural language processing and text generation is ChatGPT’s large language model (LLM). Aside from the stunningly sophisticated content it creates (photography, poems…even scientific theses!), it is its simple conversational interface and human-like reasoning that skyrocketed it to success. ChatGPT managed to make AI accessible to anyone and everyone almost overnight.
While LLMs are certainly at the forefront of generative AI news, enterprises are quickly finding that they are not the answer to fundamental business planning use cases which depend on enterprise-specific, tabular and time series data. For this, we’re seeing new generative AI options emerging, such as the Large Graphical Model (LGM) which is purpose-built to address the unique characteristics and requirements of enterprise data.
...enterprises are quickly finding that [LLMs] are not the answer to fundamental business planning use cases...
How do Large Language Models compare to Large Graphical Models?
Though Large Language Models (LLMs) and Large Graphical Models (LGMs) both fall into the Generative AI category, they are structurally very different and serve unique purposes. LLMs primarily work on text, using sophisticated algorithms and neural networks to process and generate language. They are trained on publicly-available, internet-scale text data, allowing them to learn patterns, context, sentiment, and relationships within the data to answer questions and create new text and images.
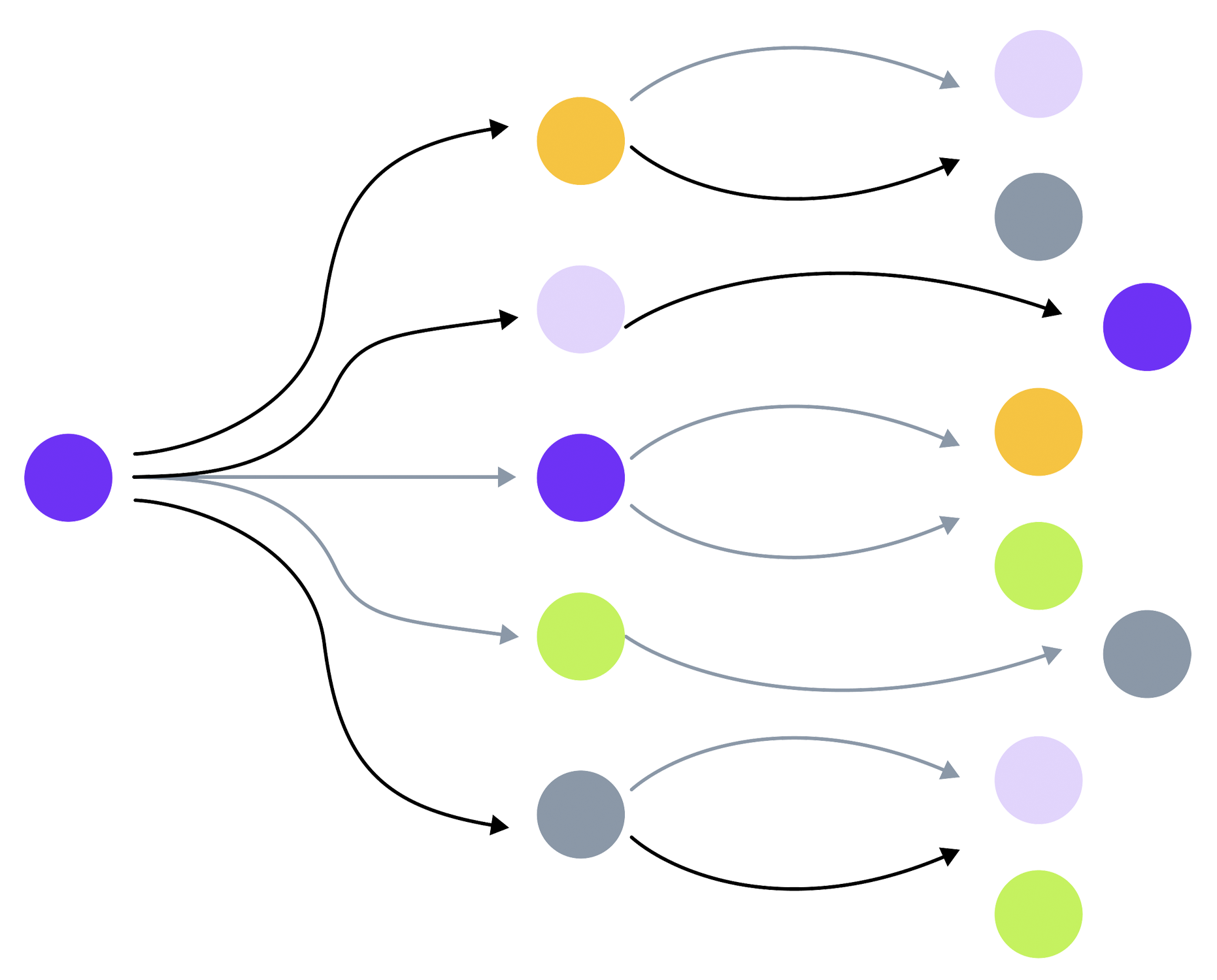
LLMs analyze text documents in a linear fashion, typically left to right, top to bottom, whereas LGMs are designed to find and evaluate relationships across multiple dimensions. This makes LGMs highly effective for tabular and time series data, which is by nature multivariate and multi-dimensional (aka, non-linear). Simply stated, an LGM is a generative AI model that produces a graph to represent the conditional dependencies between a set of random variables. The goal is to create connections between the variables of interest in order to find relationships, create synthetic data, and predict how the relationships may change in the future based on various conditions.

Data input
One major differentiator of a Large Language Model is that it primarily leverages publicly-available data to train its models, such as Wikipedia entries, articles, glossaries, and other internet-based resources. This lends itself well to use cases such as creating marketing content, summarizing research, creating new images, and automating functions such as customer support or training via chatbots.
...the Large Graphical Model is designed for enterprise-specific or proprietary data sources...
In contrast, the Large Graphical Model is designed for enterprise-specific or proprietary data sources, making it highly effective for business use cases that depend on an organization’s historical data. For example, forecasting the demand for a product may require looking at past orders, marketing promotions, discounts, sales channels, and product availability, and projecting that into the future – sometimes with different assumptions, such as a new supplier.
Computational differences
The drive to improve the power and accuracy of LLMs has led to a massive increase in the number of parameters that the models must evaluate. More parameters enable greater pattern recognition and calculation of weights and biases, leading to more accurate outputs. As such, LLMs have gone from evaluating millions of parameters to evaluating billions or even trillions of parameters in the space of a few years. And all of this comes at the growing cost of network resources, compute cycles, and energy consumption, making it cost prohibitive for all but the deep pocketed enterprises to enable LLMs at enterprise scale.
LGMs, on the other hand, are more computationally efficient and require significantly less data to generate precise results. By combining the spatial and temporal structure of data in an interactive graph, LGMs capture relationships across many dimensions, making them highly efficient for modeling tabular and time series data. In contrast to LLMs, the LGM model was found to deliver over 13X faster scientific computation on a commodity laptop compared to modern infrastructure using 68 parallel machines. This makes LGMs accessible to businesses of all sizes and budgets.
...the LGM model was found to deliver over 13X faster scientific computation on a commodity laptop...
Privacy and security
Privacy and security are a top concern for organizations interacting with commercial LLMs over the internet. A 2023 survey by Fishbowl found that 43% of the 11,700 professionals who responded say they are using AI tools for work-related tasks, raising concerns that proprietary data may be finding its way onto the internet. Since LGMs are designed to work with enterprise data, they are governed by an organization’s own privacy and security policies and do not pose the same concerns for data leakage or hallucinations that LLMs do.
Common use cases for LLMs and LGMs
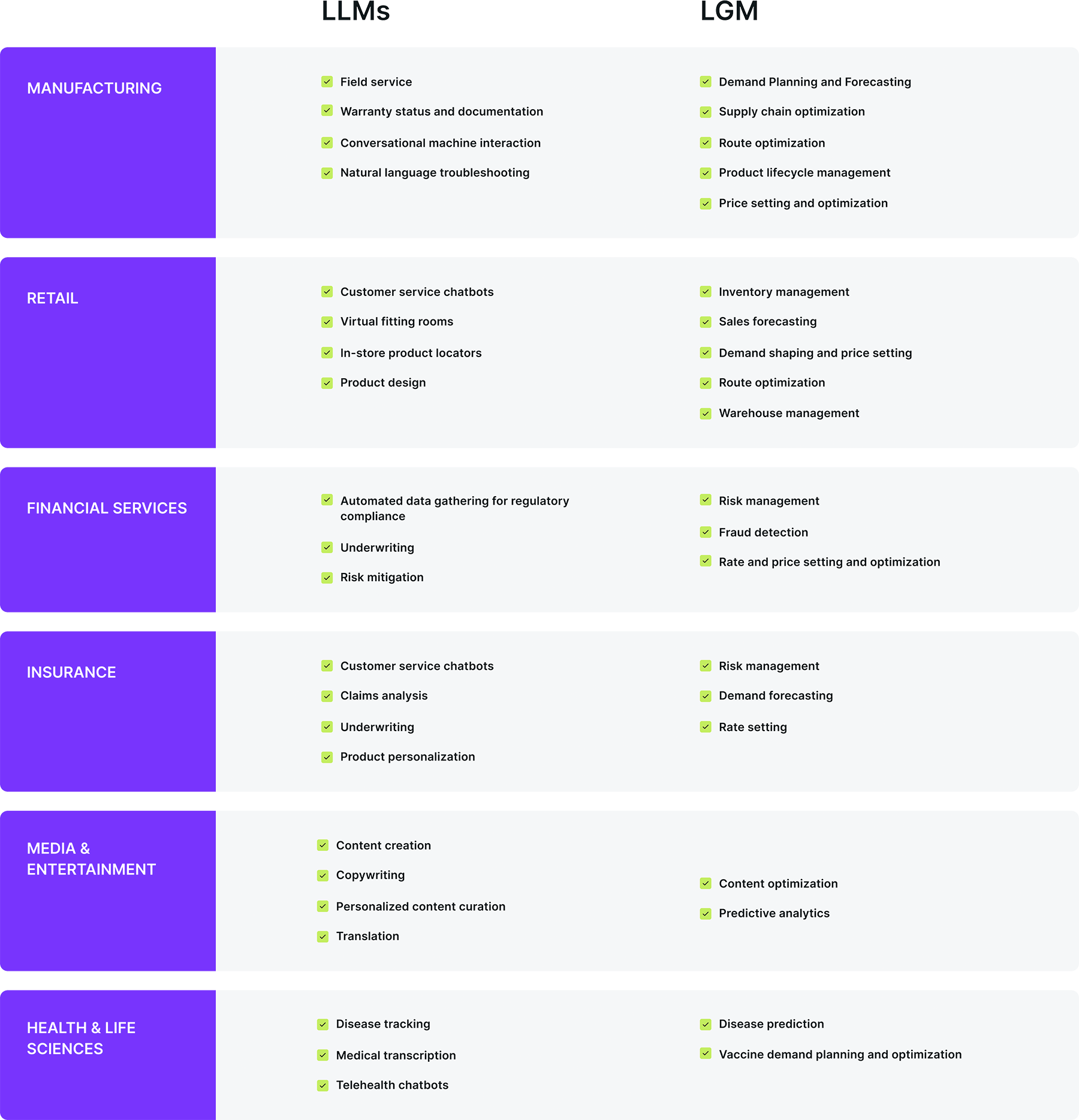
Enterprise challenges with Generative AI
Understanding enterprise data
What’s unique and different about enterprise data? The reality is that most enterprise data, such as order information, vendors, customers, and employees, is tabular and timestamped, making it a vital resource for insights into past performance, trends, and seasonality, as well as predictions about the future.
The challenge for enterprises is that tabular and time series data is typically sparse. As opposed to the internet-scale data that LLMs ingest, enterprise data can be limited, not maintained at the right level of granularity after a few weeks or months and is often distributed across many internal and external data sources. For example, consider a retailer trying to predict demand for a new product or a manufacturer performing labor planning for a new site. With limited historical data to work with, typical means of forecasting and planning fall short.
The ability to connect historical data with external data sources greatly improves forecasting and planning accuracy...
Compounding this problem is the fact that historical data alone doesn’t provide an accurate enough prediction of future behavior. In the dynamic world we live in there are seemingly endless factors to consider - weather, geopolitical factors, promotions, interest rates, and social behavior are just some of the real-time influences that business planners need to consider when forecasting demand and planning. The ability to connect historical data with external data sources greatly improves forecasting and planning accuracy by predicting how different real-time factors may influence future behavior.
How to set your business up for Generative AI success
1. Select the model that's right for your use case
Different use cases may require different models. As previously covered, Large Language Models are well suited for text-based tasks like content generation while Large Graphical Models are designed for numeric, timestamped functions such as demand forecasting and scenario planning.
2. Identify internal data sources
Internal data sources include proprietary datasets and enterprise-specific data within an organization’s security perimeter. Enterprise data is typically found in spreadsheets, databases, and data warehouses and include operational data about your employees, customers, sales, inventory, financials, marketing operations, and more.
3. Identify external data sources
Most business planning activities benefit by enriching internal data with external data. Identify which external sources influence your decision-making, such as competitive data, social media, holidays, or macroeconomic conditions.
4. Assess available data
Some generative AI models like LLMs require massive amounts of data to learn relationships and patterns to generate meaningful outputs. Other models such as LGMs operate well on sparse data. Ensure that you have access to the right types and quantity of data to generate the outcomes you need.
5. Evaluate data quality
Good AI outcomes depend on high quality data. For best results, run a data analysis tool against your source datasets to understand data gaps and inconsistencies, as well as the spatial and temporal structure of your data. This will establish a baseline of your data health and assist in resource planning for data cleansing, which often accounts for as much as 80% of a business planner’s time.
6. Cleanse and normalize data
Once data assessment is complete, leverage AI to cleanse and stitch together datasets into a single aggregated data source. In this step, the model will learn relationships between variables to match similar columns, synthesize data to fill gaps, and normalize fields for consistency.
7. Incorporate domain-specific knowledge
AI-powered outcomes are significantly more accurate and relevant when domain expertise, intuition, and experience are incorporated into model training. For each AI use case, identify key domain experts and build in automated feedback loops to review, accept, reject or adjust predictions, to continuously improve model accuracy.
8. Build in explainability
For AI outputs to be trusted and adopted by business decision-makers, they need to be easily understood and explained. Incorporate explainability into AI outputs so business users can easily understand why certain predictions or actions were made, incorporating factors such as confidence intervals, trends, seasonality, anomalies, and external influences.
9. Make AI accessible to business users
Evaluate the current apps and methodologies in use by business users and formulate a plan for successful AI adoption. Siloed AI models have low adoption rate amongst business users that typically utilize spreadsheets or integrated business systems, therefore, business leaders must prepare their workforce for change, deliver AI outcomes in ways that are easily consumable and integrated into current business practices, and equip them with the right skills to adopt and execute in an AI-powered workplace.
The Ikigai Platform Explained
Ikigai: A new approach to generative AI
Ikigai is a Generative AI platform that transforms how businesses handle internal and external structured data, particularly time-stamped information, turning it into actionable insights. Grounded in award-winning MIT research, Ikigai’s Large Graphical Model (LGM) powers the platform’s aiMatchTM, aiCastTM, and aiPlanTM models and industry solutions. These enable tabular and time series data reconciliation, forecasting, outcomes-driven scenario planning, and outcome optimization.
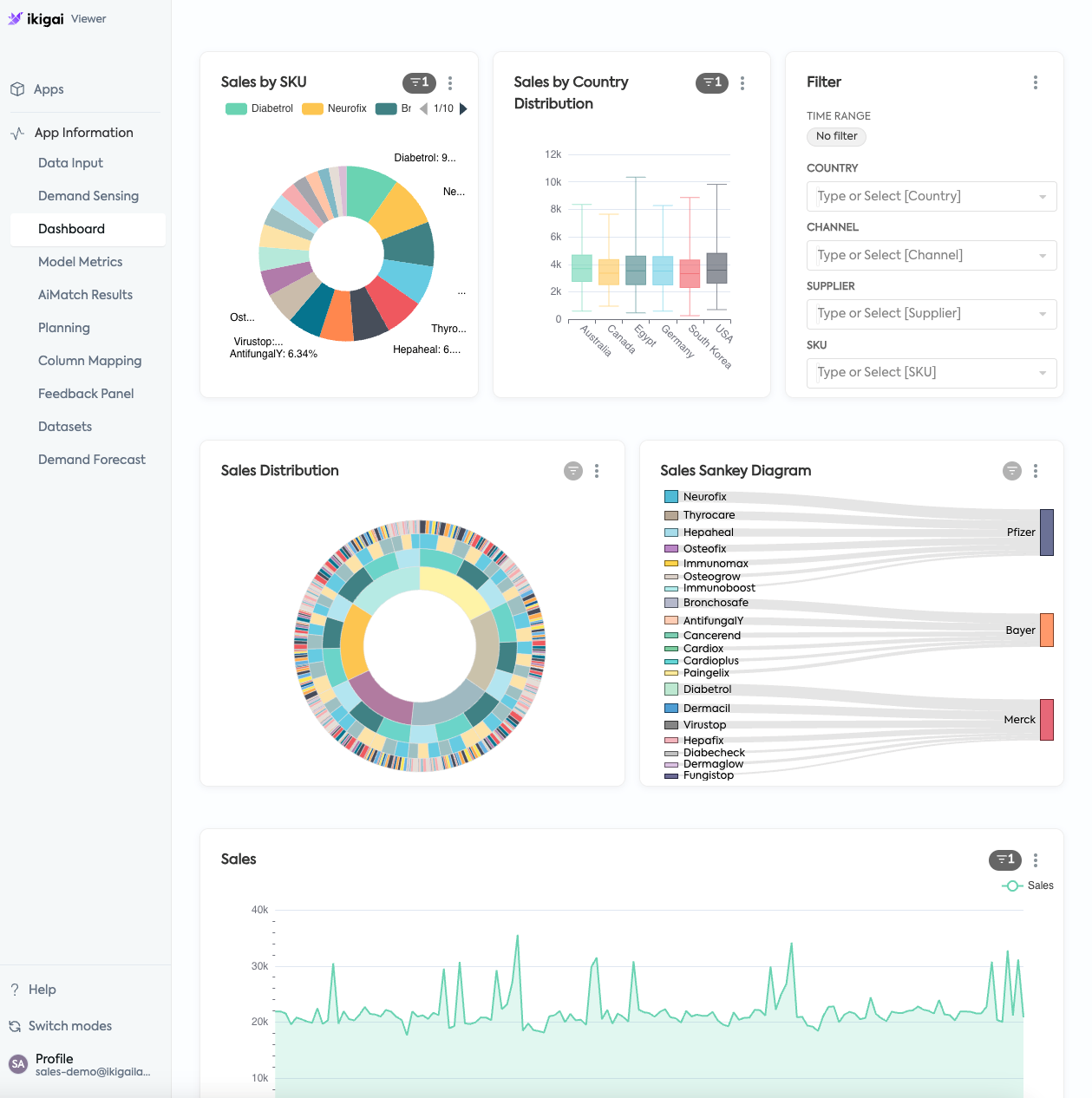
The Ikigai platform is compatible with more than 200 data sources, from spreadsheets to data warehouses to ERP platforms, and offers freedom of cloud deployment choice. Ikigai empowers business users to adopt an AI-powered approach to forecasting and planning by providing pre-built industry-specific solutions as well as an intuitive drag-and drop user experience for model building. The platform also provides a powerful AI developer environment with API toolkits and a low code/no code experience for rapid prototyping.
The eXpert-in-the-loop (Xitl) is a core capability of the platform, blending human expertise with the power of AI. It is a vital bridge between data-driven insights and real-world decision-making, enabling domain experts to train and improve the AI models with industry-specific knowledge and intuition. This fusion of human expertise and artificial intelligence empowers more informed, context-aware choices, driving greater competitive advantage and profitability.
The Ikigai platform can be utilized as an end-to-end solution for reconciliation, forecasting, and scenario planning across any industry use case, and easily integrates with existing systems via APIs.
- Connects and reconciles data in seconds
- Forecasts on as little as 2-3 weeks of data
- 20% more accurate than a dedicated tam
- Runs up to 1019 planning scenarios
The Ikigai platform
The Ikigai platform is an end-to-end platform that enables business users and data scientists to work seamlessly on one platform, leveraging the power of Large Graphical Models to connect and reconcile enterprise data sources, forecast business outcomes, and perform what-if scenario planning.
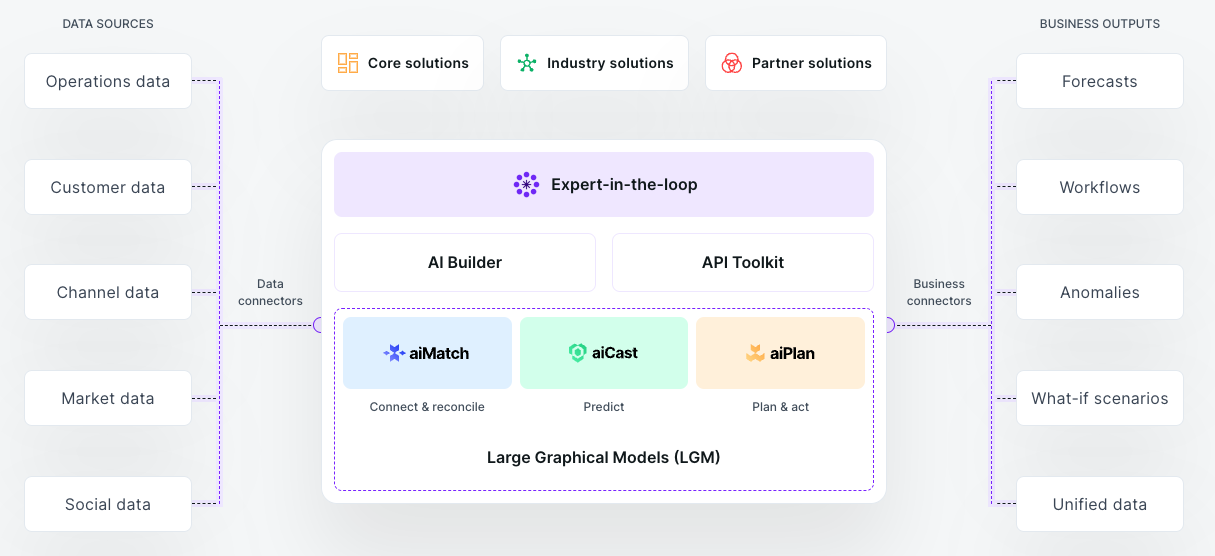
Data reconciliation with aiMatch
Data: Too much of a good thing?
You’ve probably heard that more than 80% of a data analyst’s time is spent in data preparation, leaving only 20% of their time to analyze and derive insights from the data. The reality is, the 80-20 stat has remained the same for years. So why isn’t this getting any easier?
For one, the data required for comprehensive insights and decision-making typically comes from a variety of data sources that span multiple locations and systems of record. And despite advancements in AI and an abundance of data management applications, reconciling data still requires a high degree of manual effort from domain experts - identifying and connecting the correct data sources, classifying, cleansing and normalizing the data.
And the problem is just getting tougher as exponentially more data is being generated by sensors, devices, feeds, and edge locations.
80% of an analyst's time is spent in data preparation
A poor data foundation can have a significant impact on your business – both financially and with respect to brand reputation.
- Missed revenue opportunities due to inaccurate or missing customer, order, inventory, or shipment data
- Reduced efficiency from time-consuming manual data reconciliation
- Poor customer experience caused by data inaccuracies, lack of meaningful insights or personalized content
- Inaccurate decision-making due to poor data quality, sparse historical data, and incomplete data inputs
- Increased risk resulting from data inaccuracies, lack of compliance, and poor data reconciliation practices
Data reconciliation with aiMatch
Ikigai leverages its Large Graphical Model technology to automate data reconciliation so analysts can spend more time deriving insights from their data than wrestling with it.

Connects and combines multiple data sources
Provides seamless access to over 200 enterprise and external data connectors for faster time to value
Performs feature engineering
Learns relationships between columns to identify the most significant variables, improving model performance
Imputes missing data
Fills in gaps to enable accurate forecasting and planning with sparse data
Embeds domain expertise
Integrates human intuition and expertise with eXpert-in-the-loop to quickly address anomalies and exceptions for reinforcement learning
Forecasting with aiCast
Understand the past; predict with confidence
To make optimal business decisions, organizations need to be able to accurately predict future behavior based on historical performance as well as real-time demand drivers. And one of the most important trends for any business is the demand for its products and services.
In a nutshell, demand forecasting is the process of making predictions about how much of a given product or service will sell, where and when. With an accurate demand forecast in hand, businesses are better equipped to make informed decisions about inventory management, capacity planning, supply chain operations, cash flow, profit margins, new offerings, channels and more.
Even small errors in demand forecasts can translate into millions of dollars for a large enterprise. Given this, AI-enabled demand forecasting is rising as a top priority for many enterprises. According to Gartner, 45% of companies are planning to use AI-powered demand forecasting within two years.
The benefits of AI-powered forecasting
Lower costs
Highly accurate demand forecasts give planners the information they need to optimize across the business. For example, organizations can optimize inventory to reduce carrying costs, markdowns, and breakage. Supply chain operations, transportation and labor can be better aligned to demand, saving unnecessary costs and fees. As well, sales and marketing efforts can focus their time and budgets on more profitable products and services.
Increased efficiency
Replacing spreadsheets with AI-powered demand forecasting reduces time-intensive, error-prone tasks and empowers planners to focus on higher value activities like supply chain or S&OP optimizations.
Greater forecast accuracy
Machine learning algorithms learn relationships across historical multivariate time series data, synthesize missing data, integrate real-time demand drivers, and make better predictions over time through reinforcement learning.
Higher customer satisfaction
Understanding customer buying behaviors at a granular level enables businesses to plan for the right products at the right time and place, avoiding stockouts that impact customer satisfaction and brand loyalty. It also enables greater degrees of personalization, creating a more tailored, enjoyable customer experience.
Forecasting and sensing with aiCast
While there are many different forecasting apps, the vast majority of planners still rely heavily on spreadsheets. Given the critical role that forecasts play in the product lifecycle and the financial impact of an inaccurate forecast, more organizations are turning to aiCast to increase the accuracy of their forecasts.
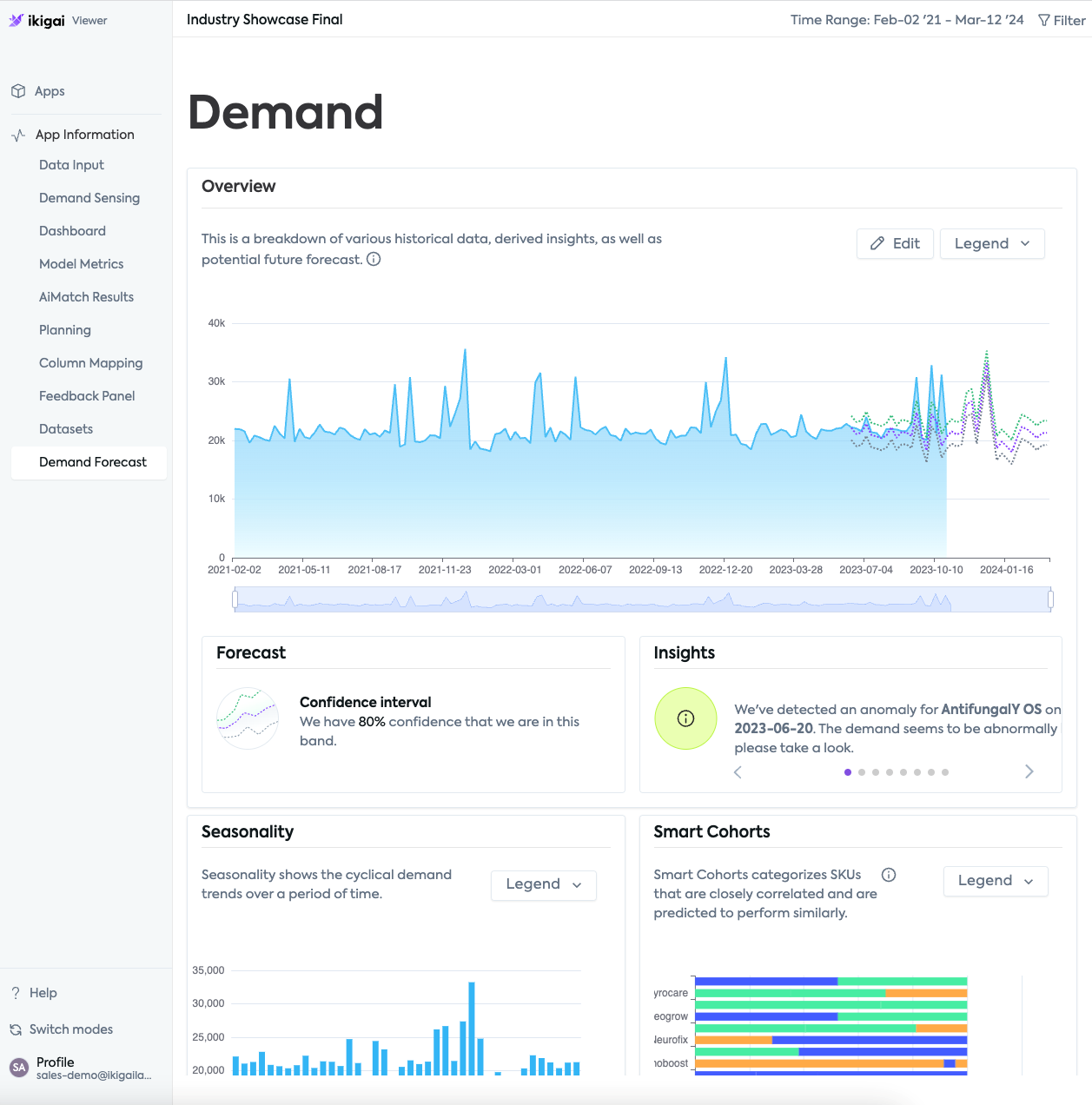
Increases forecast accuracy
Generates 20%+ more accurate forecasts – even with limited historical data
Dynamically responds to real-time data
Analyzes external data to more accurately predict demand impact and fluctuations
Delivers explainable results
Identifies anomalies and outliers, empowering domain experts to easily retrain models for greater accuracy
Enables granular predictions
Enables forecasting at detailed levels, such as SKUs, locations, categories, and more
aiCast in Action
Challenge
A large seasonal retailer faced challenges in managing its supply chain and inventory due to the seasonal nature of its products and the influence of external factors. The company needed to accurately forecast demand for a wide range of products within a very limited sales window, balancing the risk of overstocking against missed sales opportunities.
Before state
Prior to implementing the Ikigai platform, the retailer relied primarily on spreadsheets and manual processes. The resulting SKU forecasting errors led to:
- reduced sales due to stockouts
- lower margins from overstock markdowns
- high overnight shipping costs to satisfy unexpected demand
- customer dissatisfaction
Results
By implementing the Ikigai platform, the retailer was able to automate their entire demand forecasting and planning processes, leveraging aiCast to improve SKU and store-level forecasting accuracy by 34%.
34% increase in SKU and store level forecasting accuracy
Scenario planning with aiPlan
Scenario planning: The right path for your business
Scenario planning is a predictive methodology that enables organizations to explore various future scenarios, evaluate risks and tradeoffs, and plan for their potential impact. Traditional methods of scenario planning have their limitations in terms of their ability to integrate both internal and external data sources; identify relevant trends, anomalies, and outliers; generate multiple scenarios; create new scenarios based on dynamic data; and identify the optimal scenarios based on key business drivers.
With generative AI, organizations can overcome many of these challenges, using powerful machine learning models to rapidly generate and evaluate more scenarios.
Key criteria for effective AI-powered scenario planning:
- Data inputs
Identify both internal (historical) and external data sources which can influence outcomes, such as climate change, macroeconomic data, social sentiments, competitive data, or global conflicts. - Business drivers
Define relevant business constraints that should be considered in your scenario plans. This might include optimizing for cost, inventory, labor, or a potential acquisition. - Scenario generation
The more scenarios that can be generated and evaluated, the more agile and prepared your business can be for shifting strategic priorities or uncertainty. - Explainability
Ensure that scenarios include clear explanations of the strengths and weaknesses, tradeoffs, and key criteria evaluated in each scenario.
Scenario planning with aiPlan
Scenario planning has become infinitely more complex as the world around us becomes increasingly more digital. An explosion of data sources, data volumes, channels, and external influences necessitate a new AI-driven approach to scenario planning.
Ikigai’s foundational aiPlan model addresses the complexities of modern scenario planning by running thousands of AI-generated simulations against internal and external data to evaluate the impact and tradeoffs of each choice. Planners are then able to explore all possible outcomes against their unique business constraints to choose the plan that’s best for their business. They are also equipped with a variety of contingency plans in case business drivers change or unexpected events occur, such as supply chain disruptions, geopolitical events, or economic changes.
aiPlan in Action
A large meal delivery service was challenged with accurately forecasting customer support call volumes for proper staffing of their support agents. This resulted in either overstaffing, leading to unnecessary cost, or understaffing which impacted customer satisfaction.
By employing aiCast and aiPlan for demand forecasting and labor planning, the company significantly improved their labor forecasting accuracy, leading to a decrease in support costs, reduced wait times, and improved customer satisfaction. The projected annual labor cost savings is nearly three quarters of a million dollars.
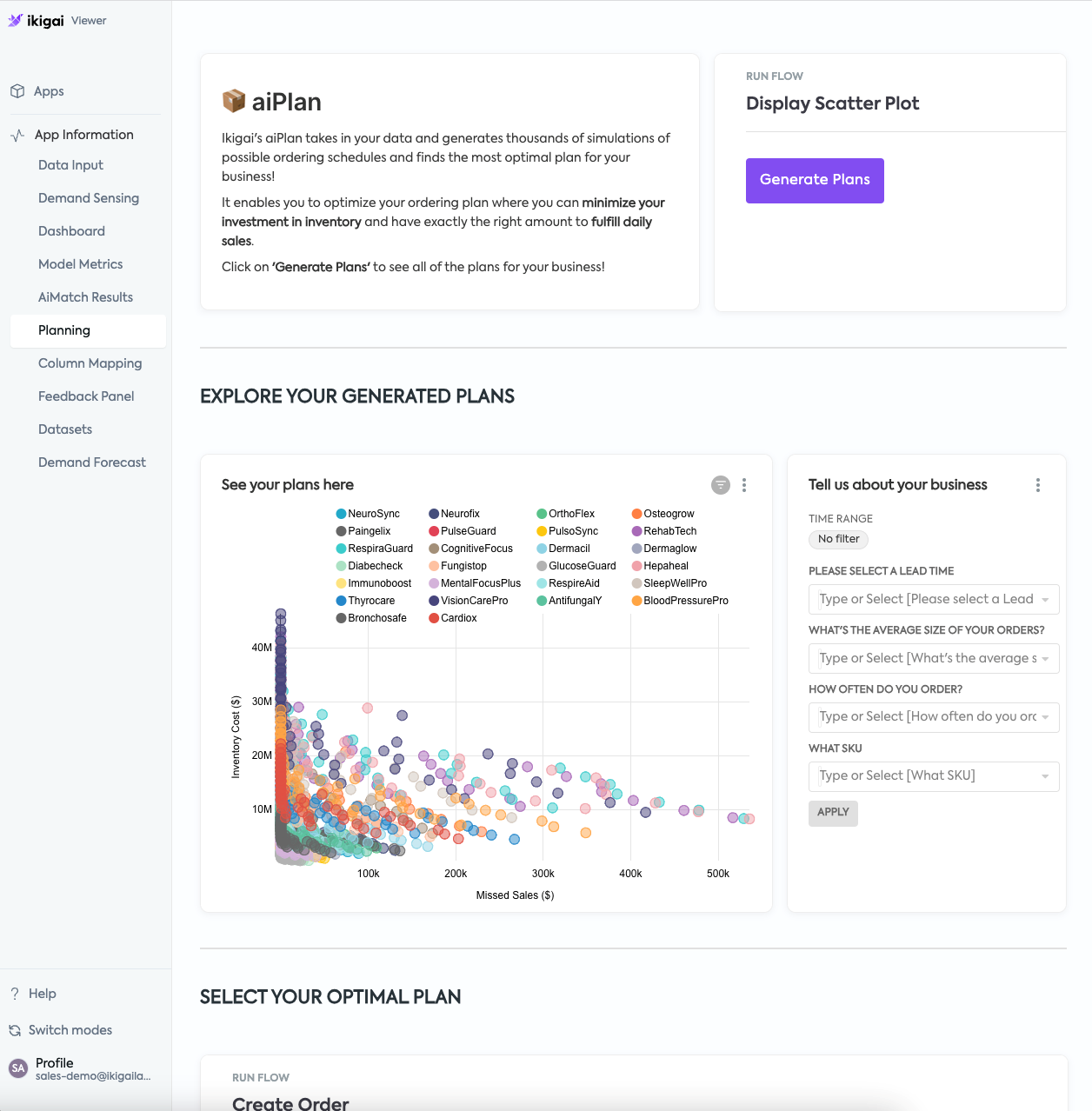
Generates 1019 scenarios
Improves quality of plans by evaluating significantly more scenarios and business constraints than other apps or methodologies
Evaluates historical and dynamic data
Incorporates both internal and external data to create more robust scenarios and enable more effective planning
Supports contingency planning
As opposed to static, rules-based systems, aiPlan dynamically generates updated scenarios based on new business drivers or unplanned events
Provides explainable results
Clearly explains decision criteria, tradeoffs, and recommendations for improved trust and adoption
The expert in the loop (Xitl)
While AI can sometimes be eerily human-like, it will never possess human intuition and real-world experience. It’s only as good as the data and the models that feed it and without human intervention, it is incapable of interpreting various nuances in trends and patterns that come with deep domain expertise, intuition, and reasoning.
To address this challenge, Ikigai has adopted an accountability-first approach, allowing users and experts to review, accept, reject, or adjust AI predictions. The eXpert-in-the-Loop (Xitl) is a core feature of the Ikigai platform. Human decisions are then integrated into model updates, enhancing future outcomes. This not only promotes transparency but also ensures that the insights provided are in sync with domain expertise.
Ethics in AI
Ethical and responsible innovation in generative AI is one of the leading issues for enterprises and the tech industry, underscored by multiple high-profile lawsuits and recent government decisions, including the EU’s AI Act and President Biden’s executive order. A multitude of ethical challenges have emerged in AI development, ranging from bias and discrimination to concerns over privacy and security.
Ethical considerations should be integrated into the entire AI lifecycle – from initial strategic decisions to development and deployment.
Data privacy – Robust privacy measures, such as consent, anonymization, and data minimization should be utilized by AI algorithms to protect individuals, groups, and companies
Bias – Bias detection algorithms should be employed to avoid unfair representation or treatment of individuals or groups due to race, gender, or socioeconomic status
Explainability – Clear explanations of how AI outputs were derived must be made available to the end users to establish trust and improve adoption
Security - All measures should be taken to protect against malicious attacks, improper use of data, and harm to individuals, groups, or companies
Compliance – Organizations must ensure that AI deployments comply with relevant laws, such as GDPR, HIPAA, and CCPA
Ikigai is committed to AI for good and recently launched an AI Ethics Council with leading academia and entrepreneurs representing diverse experience in the fields of data science, data-driven decision-making, social systems, AI and ethical algorithms, law and national security.
Getting started with Ikigai
Data understanding
Assess data complexity, context, and dependence
- What type of data is available for this use case?
- Is it primarily numerical and time-series based?
Understand context and granularity of data required
- What is the data format?
- What specific features need to be analyzed?
Goals and context
Identify core objective and outcome
- What is the problem you’re trying to solve with this use case?
- What kind of predictions or risk management are you trying to accomplish?
Define time horizon and suitability
- What is the desired time horizon for your predictions? For example, real-time/short-term (days/weeks) or long-term (months/years)?
Evaluate context and potential limitations
- What other factors or domain knowledge influence this use case?
Decision making and transparency
Understand decision support role and execution requirements
- How will you use LGM-based predictions to inform your decision-making process?
- Will this be the sole basis for action?
- Where will the action be executed (what system or process within the function)?
Assess need for interpretability and explainability
- Is it important to understand how the model arrives at its predictions?
- Is the accuracy of the results sufficient?
Other considerations
Address potential regulatory or ethical concerns
- Does this use case require any sensitive data or personal information?
- How will the privacy and ethical usage of this data be ensured?
Ensure responsible AI practices
- Are there any potential biases in the input data or model that could impact the fairness of the predictions?
Conclusion
Generative AI is unlocking new opportunities for enterprises to gain operational efficiency, new business insights, and drive competitive advantage. But navigating the evolving AI landscape requires leaders to make informed, strategic decisions about where and how to employ generative AI in their business.
Use cases such as sales and operations planning, supply chain, and workforce management all rely on data reconciliation, forecasting and scenario planning - usually with complex data and a high degree of uncertainty. And while the business outcomes differ, they all depend on tabular, timestamped data that run your business - your people, products, budgets, and operations.
The Ikigai platform was built on the foundation of patented Large Graphical Models and designed specifically for tabular and time series data. It delivers greater performance and accuracy and enterprise scalability - all at significantly lower cost than traditional AI models. The platform brings flexibility to your AI solution building by offering a powerful AI developer environment as well as prebuilt industry solutions and a simple drag-and-drop, low code/no code experience for business users.