Eliminating Guesswork in New Product Launches: How Ikigai’s AI Redefines Demand Forecasting
Last update: February 13, 2025
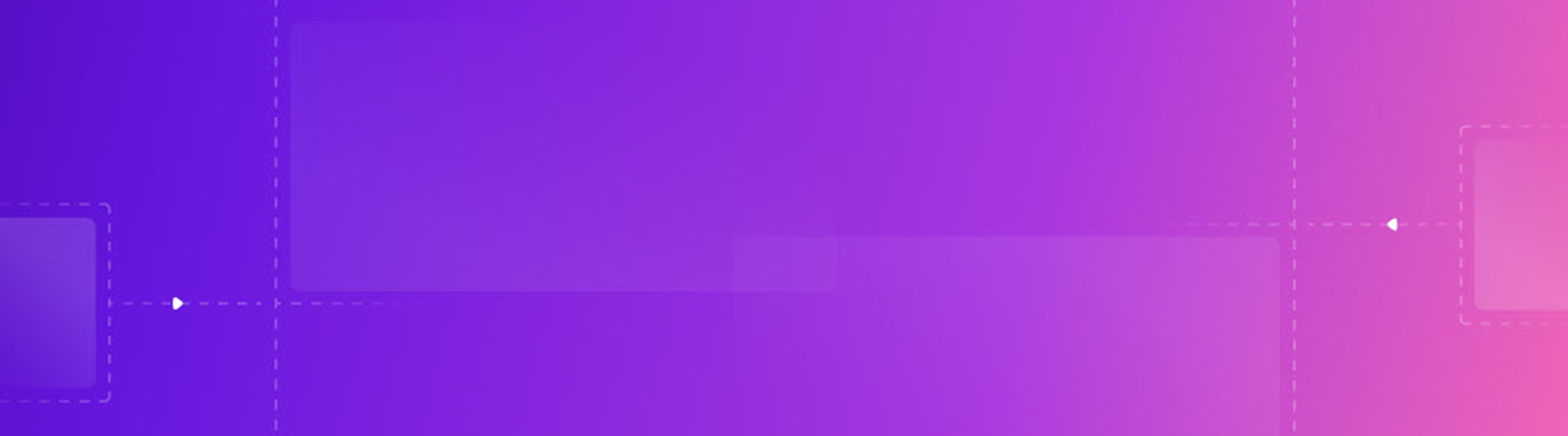
The New Product Dilemma
New Product Introductions (NPI) are inherently high stakes. Whether you’re rolling out a groundbreaking innovation, an iterative version, or a complementary product or solution, predicting demand accurately is crucial to avoid revenue loss, brand damage, and operational bottlenecks. Traditional forecasting methods— rooted in manual spreadsheets or simplistic models —often fall short in today’s dynamic, data-rich environment.
Every new product launch carries a significant amount of uncertainty and risk given the lack of available historical data to adequately predict future demand. In fact, The Institute of Business Forecasting & Planning (IBF) reports an average forecast error rate of 64% for new products.
New products have an average forecast error rate of 64%.1
Ikigai’s AI-driven Demand Forecasting solution offers a proven alternative, using advanced modeling techniques specifically tailored for new products — even when no historical data exists. This guide explores common pitfalls of new product forecasting, demonstrates how Ikigai addresses them with its modern AI-based approach, and provides executive-level ROI insights. It also answers practical questions about how to incorporate AI forecasting into critical business processes to maximize market potential for every product from launch to end of life.
The Challenges of New Product Introduction Forecasting
Introducing a new product to the market presents unique forecasting hurdles. From the absence of historical data to multi-level complexity across regions and channels, these challenges can quickly erode profitability and damage customer trust. Understanding these critical pain points—and the shortcomings of legacy processes—forms the foundation of a robust NPI forecasting strategy.
Some common challenges include:
.png)
Why Traditional Tools and Approaches Fall Short
Many enterprises still rely on spreadsheets or rigid deterministic systems that are simply not set up to manage the complexities that launching a new product involves. Now that we’ve outlined the core new product forecasting challenges, these methods:
Suffer from rigid historical dependence:
Spreadsheets and deterministic ERP modules generally need robust historical data for baseline forecasts.
Lack external data integration and flexible modeling:
They either ignore or are unable to incorporate relevant external signals and they can’t adapt to brand-new product attributes or external signals, leaving teams to make manual adjustments that are slow and prone to error.
Can’t adapt quickly:
Single-point estimates fail to capture the real-world volatility of NPI demand.
Struggle with global complexity:
Coordinating forecasts across multiple geographies or SKUs is cumbersome and error prone.
Don’t foster cross-functional collaboration or insight:
Data remains siloed, preventing unified decision-making.
An AI-Driven Approach to New Product Forecasting
The shortcomings of traditional tools — manual data handling, static models, and poor cross-functional visibility — underscore the need for a modern forecasting solution. In this section, we’ll see how Ikigai empowers organizations to eliminate guesswork and confidently launch new products in dynamic markets.
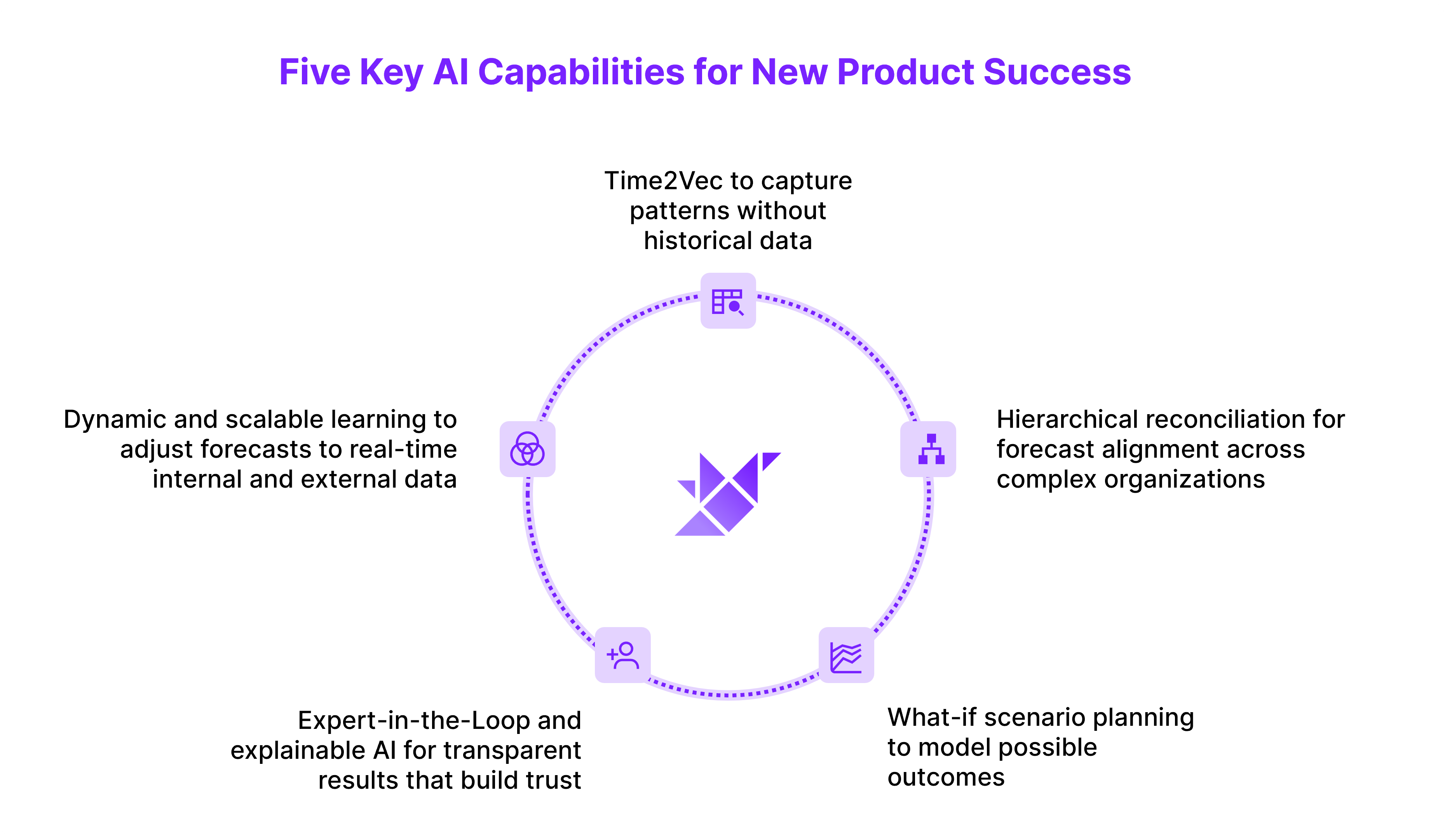
Explore Ikigai's interactive demo on new product forecasting here.
Time2Vec
.png)
Time2Vec is a unique Ikigai algorithm that learns temporal patterns (seasonality, trends) from analogous products, competitor data, and external signals. For new products lacking direct sales history, Time2Vec infers likely demand patterns by analyzing similar channel, region, product histories and attributes, alongside external data factors.
How it works
Unlike traditional methods that rely on manually identifying similar products and determining how to incorporate relevant periods of their history, Time2Vec automatically identifies the most relevant products based on both historical performance data and detailed product attributes like descriptions and specifications.
Furthermore, the algorithm uses a sophisticated approach that weighs and combines multiple product histories. For example, it may determine that the new SKU is 30% similar to Product A, 20% similar to Product B, and 50% similar to Product C, creating a more accurate forecast based on the composite of three similar products.
Time2Vec also intelligently selects the most relevant time periods from each product's history, rather than using all historical data indiscriminately. For example, instead of using a product's entire sales history, it might determine that a specific three-month period provides the most relevant data for predicting the new product's performance.
This selective approach to both product similarity and temporal relevance enables Time2Vec to create more nuanced and accurate demand forecasts for new products without direct sales history.
Probabilistic Modeling and ‘What-if’ Scenarios
.jpg)
Instead of static forecasts, Ikigai produces probability distributions that show a range of possible outcomes, letting you explore best-case and worst-case scenarios. Companies can model the impact of pricing changes, promotional campaigns, or supply constraints before they occur.
How it works
Ikigai's AI-driven scenario planning transforms traditional forecasting by generating dynamic probability distributions rather than fixed predictions. This approach provides a comprehensive view of potential outcomes, from optimistic to pessimistic scenarios, enabling businesses to better understand and prepare for various future possibilities. Instead of relying on a single forecast number, decision-makers can see the full spectrum of likely outcomes and their associated probabilities.
The platform's sophisticated Time2Vec model forms the foundation of this capability by learning complex relationships between variables in your dataset and external factors. When you modify any variable - whether it's pricing, promotional timing, or supply chain parameters - the model dynamically recalculates the entire probability distribution.
This means you can instantly see how changes in one area ripple through your business, affecting everything from demand patterns to inventory requirements. For example, you could model how a 10% price increase in marketing spend might impact demand across different regions, or how a major promotional campaign could strain your supply chain.
Hierarchical Forecasting with Global Reconciliation
Ikigai aligns forecasts across products, SKUs, regions, and channels, ensuring consistency from the local store level up to global views - key for large, multi-regional operations managing seasonal or region-specific demand.
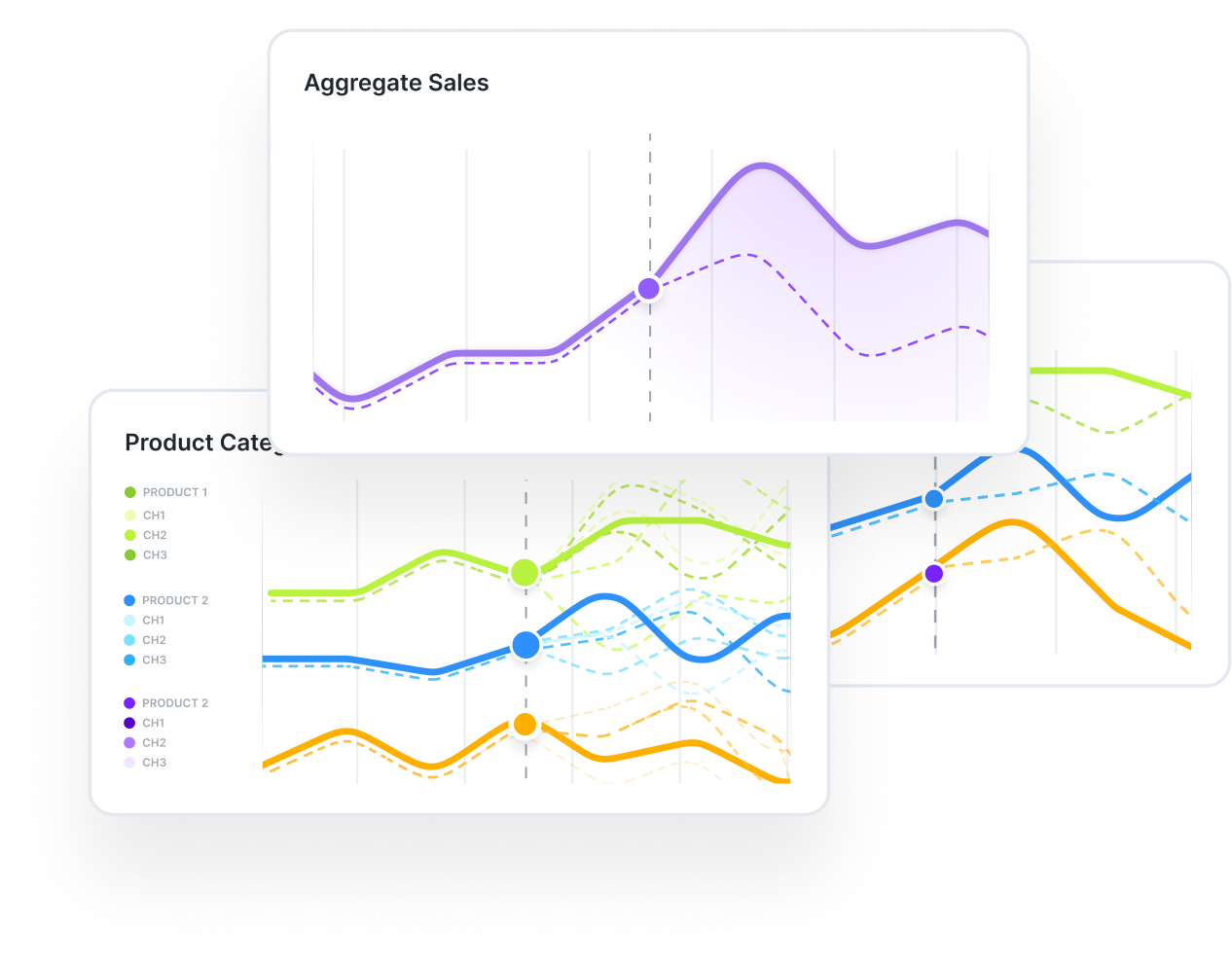
How it works
To ensure forecast consistency across all levels of business operations - from individual SKU performance to corporate views - Ikigai utilizes advanced hierarchical reconciliation techniques. The platform intelligently aligns predictions across products, SKUs, regions, and sales channels, creating a cohesive forecasting framework that maintains accuracy at every level of the hierarchy. This is particularly valuable for large organizations managing complex, multi-regional operations where seasonal demands and regional preferences can vary significantly.
Ikigai’s hierarchical reconciliation goes beyond simple forecast alignment by leveraging data relationships across product categories, channels, and regions to provide deeper insights into product performance dynamics.
When introducing new products, the platform can predict potential sales cannibalization effects on existing product lines while also identifying opportunities for complementary sales growth. For example, the system might detect that while a new premium product variant could decrease sales of a mid-tier option, it might simultaneously drive increased sales of complementary accessories or related products. This comprehensive understanding of product interactions enables businesses to make more informed decisions about product launches and portfolio management.
Expert-in-the-Loop (XitL)

AI plus human domain expertise drives better outcomes. Domain experts like supply chain managers can override or refine AI outputs based on market knowledge or strategic considerations (e.g., a pending competitor launch). This creates trust in AI-driven insights and enhances cross-functional collaboration.
How it works
To deliver more accurate and actionable forecasts, Ikigai's expert-in-the-loop (Xitl) approach combines the power of AI with invaluable human domain expertise. The platform enables supply chain managers and other domain experts to directly incorporate their market knowledge and strategic insights into the AI-generated forecasts and plans.
Whether it's awareness of an upcoming competitor launch, anticipated market shifts, or other factors that may not be captured in historical data, experts can refine and override AI predictions to ensure forecasts reflect the complete picture of market dynamics.
The Ikigai platform offers multiple levels of forecast adjustment to accommodate different types of expert knowledge. Users can fine-tune the system's understanding of product similarities, modify demand predictions for specific products, or adjust broader category-level forecasts across regions and channels. When experts make these adjustments, Ikigai doesn't simply override the AI predictions - instead, it re-simulates scenarios incorporating the new information, creating a true collaboration between human insight and machine learning. This iterative process not only produces more accurate forecasts but also builds trust in the AI system as users see how their expertise enhances the final outputs.
Dynamic and Scalable Learning
.png)
Ikigai ingests real-time performance data as well as internal and external data — be it weather, social sentiment, competitor sales or macroeconomic indicators—to dynamically update forecasts. By generating millions of forecasts across hierarchy levels and dynamically adjusting to new sales data, Ikigai reduces the lag between market changes and forecast adjustments, driving greater accuracy in existing and new products.
How it works
As opposed to traditional rules-based systems that rely on rigid, predefined logic, dynamic forecasting continuously evolves by ingesting and analyzing both real-time performance data and a diverse array of external signals. The platform processes everything from internal sales metrics to external factors like weather patterns, social media sentiment, competitor performance, and macroeconomic indicators. This comprehensive data integration enables forecasts to update automatically as market conditions shift, dramatically reducing the lag between changes in the market and corresponding forecast adjustments.
The system's learning capabilities extend beyond simple data collection to active forecast refinement based on real-world performance. As actual sales data comes in for new products, Ikigai automatically calibrates its predictions to reflect ground reality. The platform doesn't just compare forecasts to actuals - it analyzes the drivers behind any variances to improve future predictions. For instance, if a new product performs differently than expected in certain weather conditions or during specific promotional events, the system incorporates these insights into its ongoing forecasting models.
Realizing the ROI for AI-Driven Forecasting
For Supply Chain and Planning executives, one of the most pressing questions is: What is the financial payback of AI? Below are some common success metrics realized by Ikigai clients.
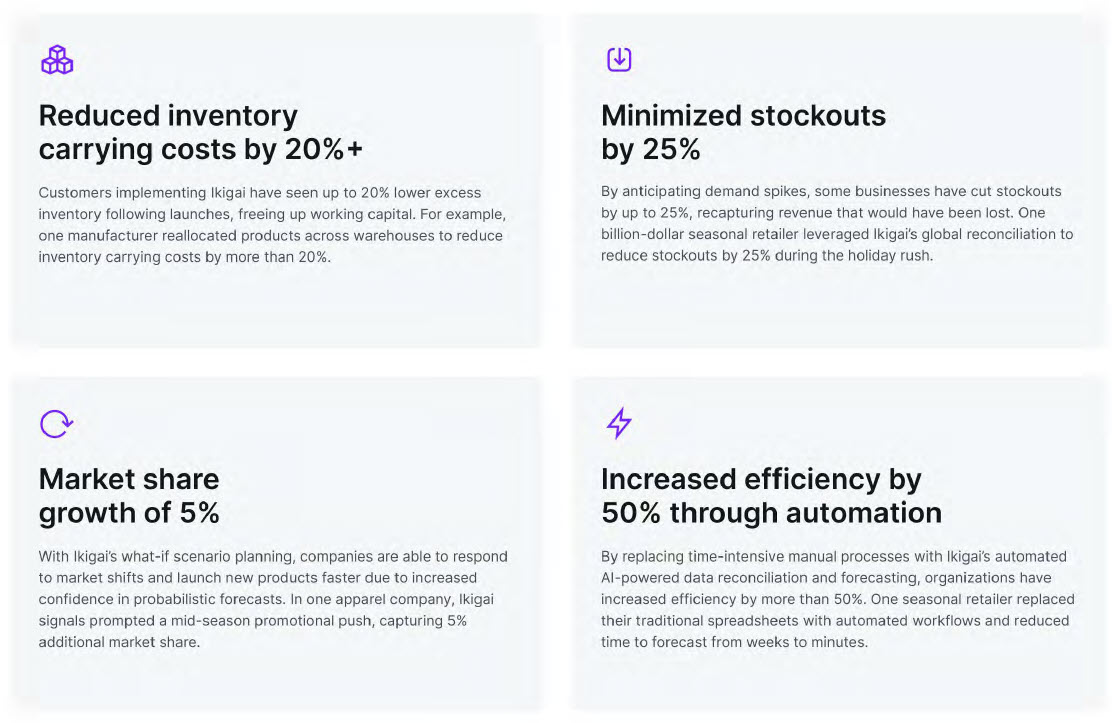
Is Your Forecasting Approach Ready for Modern NPI Challenges?
Self-Assessment
Use the quick quiz below to gauge whether your current demand forecasting strategy aligns with the complexities of New Product Introductions (NPI). Mark the option that best represents your organization’s experience. Tally the points and see recommendations at the end.

Understanding your results
0 - 4 points
Your current environment seems relatively stable or predictable. Traditional forecasting methods may suffice for many cases, but staying agile is still key. AI-driven solutions can still offer efficiency gains and prepare you for sudden market shifts.
5 - 7 points
You experience moderate complexity and uncertainty in demand. Probabilistic forecasting could be a strategic advantage—especially for product lines or regions prone to variability. Evaluating an AI-driven approach to handle sporadic promotions, limited historical data for new SKUs, or seasonal surges might yield significant benefits.
8 - 10 points
Your organization faces frequent demand fluctuations and multi-layered forecasting challenges - probabilistic, AI-enhanced forecasting is recommended. Incorporating Time2Vec for zero historical data, hierarchical reconciliation for multi-level product lines, and external signal integration can help you navigate rapid market changes and avoid costly inventory pitfalls.
Next steps

By completing this assessment, you now have a clearer sense of the opportunity to add AI to your new product forecasting. Whether you're dealing with sudden surprises or constant demand shifts, embracing AI-driven techniques can dramatically reduce errors, improve inventory management, and drive long-term confidence in launch management.
Whether you're dealing with sudden surprises or constant demand shifts, embracing AI-driven techniques can dramatically reduce errors, improve inventory management, and drive long-term confidence in launch management.
Conclusion
Launching a new product or service without historical data has long been one of business’s most challenging forecasting dilemmas. With traditional approaches yielding average forecast error rates of 64%, the cost of getting it wrong has never been higher in today’s dynamic market environment.
AI-driven forecasting represents a fundamental shift in how organizations can approach new product introductions. Through capabilities like Time2Vec’s pattern recognition, probabilistic modeling, hierarchical reconciliation, dynamic forecasting, and expert-in-the-loop functionality, businesses can now navigate launches with unprecedented precision and confidence.
The results speak for themselves. Organizations implementing AI-driven forecasting are seeing inventory carrying costs reduced by up to 20%, stockouts decreased by up to 25%, and full-price sell-through improved by 15-20% — all while dramatically reducing the manual effort required for forecast generation and maintenance.
As market complexity continues to increase and product lifecycles accelerate, the ability to launch products with AI-powered precision will become a critical competitive differentiator. Companies that embrace these advanced forecasting capabilities now will be better positioned to turn the new product paradox into a sustainable advantage.
The future of new product forecasting isn’t about eliminating uncertainty — it’s about managing it with intelligence, adaptability, and precision. With AI-driven approaches, businesses can finally move beyond guesswork to confidently navigate the challenges of bringing new products to market.
Download PDF
Click here to download this content in PDF format.
Learn more by visiting our New Product Introduction solution page.