Large Graphical Models Allow Financial Services Businesses to Cash in on AI
In an industry with trillions of global transactions, where precision and planning are priorities and complex macroeconomic trends can sink a business, Large Graphical Models can help financial services institutions spot hidden patterns, forecast the future, and make better business decisions.
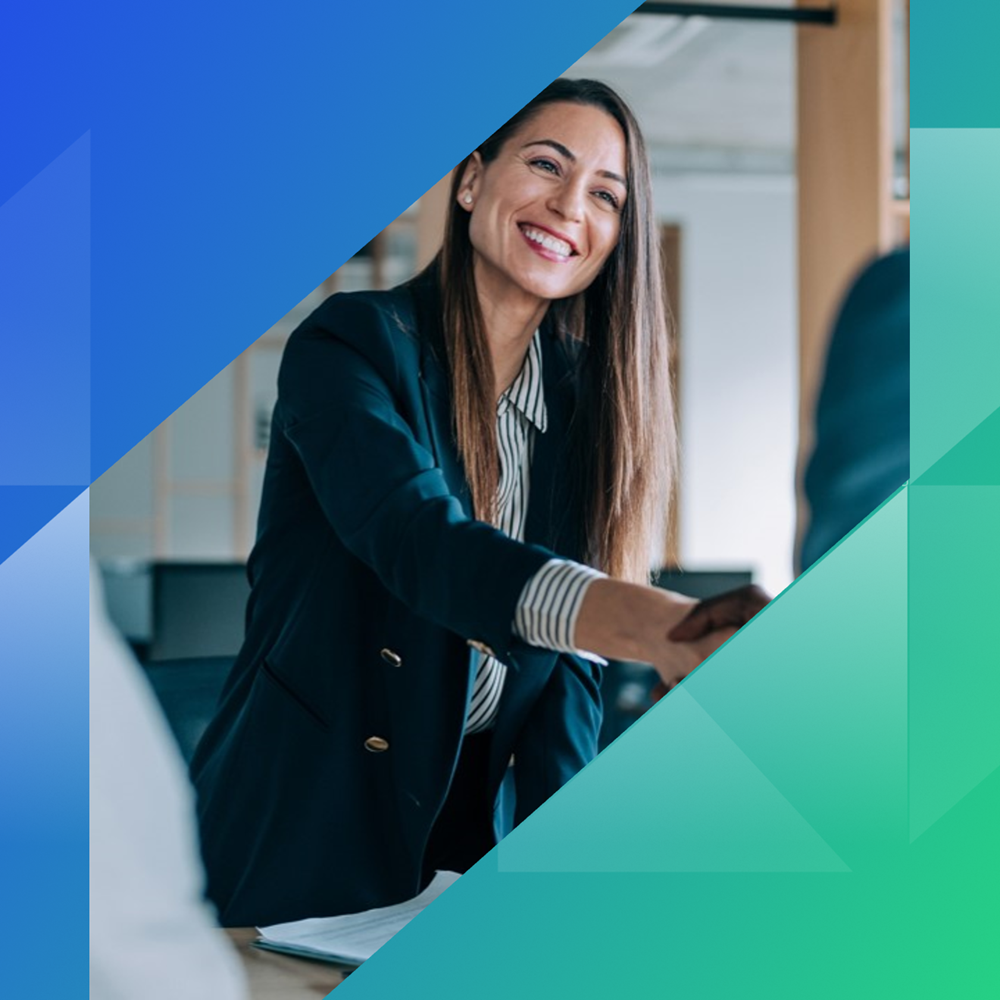
The financial services sector has evolved rapidly over past decade
Perhaps more than any other industry, the financial services sector has been transformed by major technology trends over the last decade. Shifts like growing cloud deployments, surging mobile device adoption, the consumerization of banking apps, and the proliferation of new payment methods, among others, have rapidly remade financial organizations from the inside out. These technologies have made financial institutions more efficient, created additional revenue streams, and enabled a bevy of new products and services for customers.
But while financial organizations have gained massive opportunities from these shifts, they’ve also encountered new problems. For example, these businesses now must wrangle mountains of data from many different sources, which causes major computing, networking and compliance challenges. With the rise of digital banking and numerous emerging payment options, fraud detection has also become a bigger concern. Newly released FTC data show that consumers reported losing more than $10 billion to fraud in 2023, marking a 14% increase over reported losses in 2022.
The next frontier in financial services: AI
Artificial intelligence is the latest major new technology impacting financial services. AI is sweeping across nearly every vertical, but financial services organizations– known to quickly embrace innovative tech – are near the lead in AI adoption. According to an Ernst & Young survey, “Nearly all (99%) of the financial services leaders surveyed reported that their organizations were deploying artificial intelligence (AI) in some manner, and all respondents said they are either already using, or planning to use, generative AI (Gen AI) specifically within their organization.”
In an industry with trillions of global transactions, where precision and planning are priorities and complex macroeconomic trends can sink a business, AI can help banks spot hidden patterns, forecast the future, and make better business decisions. But they need to employ the right type of AI.
The LGM opportunity
Large Graphical Models (LGMs) are a new form of generative AI that is ideal for use in the financial services sector. LGMs are a fit because they are tailor made for tabular, time-series data, which is the most common type of data in the finance industry and is essential for applications like fraud detection and financial reconciliation.
A LGM is a model that uses a graph to represent the conditional dependence structure between a set of random variables. LGMs are probabilistic in nature, meaning their purpose is to describe and explain the entire joint distribution between all variables of interest. From an end user’s perspective, LGMs produce interactive graphs that visualize the complex relationship between different data points.
As noted above, LGMs excel in modeling tabular data, which includes data typically organized in spreadsheets, tables, and databases, and time-series data, representing a sequence showcasing the evolution of a single variable over time. For instance, this might entail credit card transactions or real-time stock market changes. By approaching time-series data from the unique perspective of tabular data, LGMs possess the capability to identify patterns, uncover anomalies, and forecast broader business trends.
LGMs streamline financial reconciliation
In the world of finance, every number and figure must add up. Errors and discrepancies in financial statements can have serious consequences for institutions. Organizations undergo financial reconciliation to identify and eliminate any of these miscalculations and ensure consistency. But this process is typically time-consuming and error-prone, which limits the amount of time a financial analyst spends deriving key business insights. With LGMs, organizations can speed financial reconciliation by up to 60% and create AI-powered forecasts and plans for a wide range of purposes.
By analyzing tabular, time-series data, LGMs can:
· Ensure accuracy and consistency across financial records and statements
· Streamline transactions and invoice management
· Identify anomalies and discrepancies
· Improve reporting and compliance
LGMs support critical financial reconciliation use cases, including:
· Bank reconciliation: Ensure accuracy and consistency across financial records and statements
· AR and AP: Match payments and invoices across customers and vendors
· General ledger: Identify errors, misclassifications, and discrepancies across ledgers and source documents
· Tax reconciliation: Ensure accurate reporting and compliance with tax regulations
LGMs protect against fraud
As the digital economy expands, financial institutions face massive transaction growth, increasing regulatory controls, and rising incidences – and sophistication – of fraud. Fraud costs both consumers and banks billions of dollars, while also causing significant reputational harm. Today, financial institutions must guard against loan fraud, money laundering, wire transfer fraud, credit card fraud, ACM fraud, and new account fraud, among others.
With these high stakes, effective, modern fraud detection is a must – and LGMs provide the best way to deliver it.
LGMs are built for pattern recognition and forecasting, enabling financial institutions to reliably detect unusual activities which may indicate fraud. This provides customers with a more secure banking experience and avoids business risks to financial institutions.
Consider an example of how LGMs help thwart fraud when used for alert monitoring. Whenever an event occurs, such as a client signing up for an account or a new customer beginning to make transactions, a bank’s transaction monitoring system analyzes data about that event and sends alerts about potentially fraudulent activity to a human analyst, whose job it is to manually investigate these alerts and determine which ones require remediation. However, the vast majority (up to 95%) of these alerts turn out to be false positives. Manually analyzing all these alerts is labor-intensive, cumbersome, and often inaccurate, wasting resources and allowing for real fraud to slip through.
LGMs can be used to analyze these alerts with much greater efficiency and precision, automatically identifying patterns to determine whether an alert requires intervention from a human analyst. The tech looks at customer profile information, transaction history, and the outcomes of similar alerts from the past to make these decisions. The LGM not only weeds out false positives, but also provides explainability by giving the analyst contextual data showing why it decided that a certain event is high risk.
The result is that financial institutions – and their customers – are better protected from fraud and the organizational risks that come with it. In addition, organizations can spend fewer human hours investigating alerts and instead use those resources to create new business value.
Protect your financial institution with LGMs
Ikigai Labs’ founders created Large Graphical Models based on years of AI research and development at MIT. The company’s patented LGM technology is designed entirely for generating insights from tabular, time-series data. The tech is well-suited for financial reconciliation, fraud detection, and other use cases within the financial services space, which is overflowing with tabular, time-series data.
Dive into this whitepaper, Embracing Uncertainty: How Probabilistic Forecasting Transforms Financial Strategies, to learn new approaches to today's increasingly volatile financial markets.
Visit here to learn more about how Ikigai can help your organization wield AI to mitigate risks, solve data challenges, predict trends, and increase profitability.