Enterprise Forecasting: Navigating Uncertainty with aiCast
Last update: September 10, 2024
Introduction
Forecasting is a critical component of strategic business planning, yet decision-makers and expert systems often struggle to model the uncertainty and complexity in their operations and markets. Without understanding the "why" of historical performance across internal factors as well external variables like real world events, customer preferences or competitive behavior, accurate forecasting becomes an exercise in disappointment.
Without understanding the "why" of historical performance across internal and external factors, accurate forecasting becomes an exercise in disappointment.
Consider a global consumer goods company trying to forecast demand for a newly launched product across multiple regions. Traditional forecasting models may fall short simply because there is sparse, limited, or no historical data for that product.
What this company needs is an AI-driven approach, such as Ikigai’s aiCastTM technology, that would enable them to make full use of internal and external data, quantify risk and outcomes, and generate actionable insights key to making effective business decisions. With insights in clear view, the company and its partners can now produce, stock, and price the new product effectively, preventing overstocking or shortages.
Key Features of aiCast
aiCast is an advanced AI forecasting solution that manages the evaluation, processing, and application of forecasting models purpose-built for time stamped and tabular enterprise data. Developed by Ikigai Labs, it offers a robust forecasting approach by factoring in seasonality, trends, and interactions between complementary products. aiCast leverages advanced technology like Large Graphical Models (LGM) and Time2Vec techniques to draw highly relevant patterns from existing products and customer segments.
This paper explores the capabilities of this game changing LGM model, highlighting how it generates tailored forecasts to provide actionable insights and recommendations for improved business performance
Large Graphical Model (LGM)
At the core of aiCast is Ikigai’s Large Graphical Model (LGM), which is able to capture relationships and dependencies between various variables (ancillary multi-variate data such as weather or competitor behavior, auxiliary data such as inventory levels, and interventional data such as promotions). Unlike traditional models that handle variables in isolation, LGMs account for interconnected factors such as how the sale of one product might influence demand for another. This enables aiCast to recognize, weight and incorporate the relationships between variables, providing an integrated and accurate forecast. In the example discussed earlier, LGMs can model how the sale of a current product might affect the demand for another related product, allowing the business to develop and implement mutually advantageous inventory and pricing strategies.

Unlike traditional models that handle variables in isolation, Large Graphical Models account for interconnected factors that may influence each other.
Time2Vec: Harnessing Scarce, Limited Historical Data
One of the most significant challenges in forecasting is limited historical data. Time2Vec, an advanced technique integrated into aiCast, transforms time series data into structured embeddings that retain temporal patterns and relationships, even when historical data is sparse or inconsistent. This is particularly critical for businesses launching new products or entering new markets, where historical trends may be unavailable or insufficient. Time2Vec allows businesses to derive meaningful insights from limited data, thereby enabling decisions without losing forecast reliability.

Time Series Decomposition and Analysis
aiCast excels in time series decomposition, a critical process that breaks down time series data into its fundamental components such as trends, seasonality, and residuals while identifying and isolating unique elements like change points, errors and other anomalies. By separating these elements, aiCast can identify underlying patterns and predict future outcomes with greater precision.

Imputation: Handling Sparse, Disconnected and Missing Data
Before accurate forecasting can take place, data preparation is essential, and a key part of this process is addressing inconsistent, limited, or missing values with detection and imputation. aiCast probabilistically generates what if missing values are legitimate and if not what they might be, allowing for the creation of complete datasets.

For example, in demand forecasting, missing sales data due to technical issues in transaction records can skew the forecast. By imputing these values based on related data—such as inventory levels or nearby store sales—aiCast ensures that gaps in historical data are addressed, leading to a complete and accurate forecast. Imputation thus helps address gaps in historical records and ensures that forecasts are generated from higher-quality data.
Anomaly Detection: Reducing Errors for Better Forecasts
Another crucial step in data preparation is the identification of anomalies. These are unusual data points or errors that could skew forecasts if left unaddressed. By detecting and addressing anomalies before generating forecasts, aiCast ensures that its predictions are based on clean, reliable data. For example, outliers caused by data entry errors or system malfunctions can be corrected, reducing the risk of inaccurate forecasts.

Change Points: Adapting to Shifts in Time Series Data
Time series data often display changes (sometimes dramatic, often subtle) in behavior caused by external events. These change points, which mark where the underlying dynamics of the data shift, can significantly impact forecasting. aiCast is designed to identify these points, ensuring that models incorporate and adjust accordingly. For instance, the dynamics of online purchases changed drastically before and after the COVID-19 pandemic. Recognizing such change points enables aiCast to focus on the most relevant segments of the data for more accurate predictions.

Cohort Identification: Grouping Similar Behaviors
Within a collection of time series, some variables may exhibit similar behavior patterns. These cohorts can be critical for decision-making, as they help businesses understand relationships between different products or processes. For example, aiCast can identify cohorts of products that tend to be purchased together, allowing businesses to optimize cross-promotions or bundling strategies. Similarly, in finance, it can identify stocks or policies that behave similarly, aiding in building portfolios or market baskets based on different risk/reward profiles.
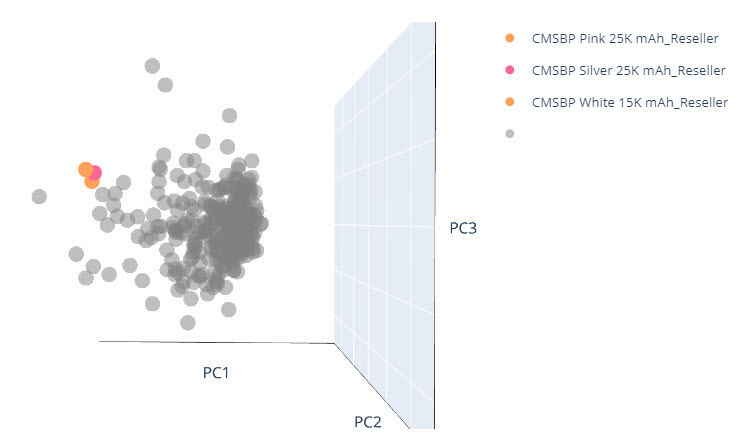
Hierarchical Reconciliation: Ensuring Consistency Across Aggregations
Many time series exhibit hierarchical temporal relationships, such as daily, weekly, or monthly aggregations. Hierarchical reconciliation ensures that forecasts are consistent across these levels. For example, the sum of daily sales should reconcile with monthly sales data. In more complex structures (spatial), such as product categories or organizational hierarchies, aiCast ensures that forecasts remain aligned across different levels of the hierarchy, preserving accuracy at both granular and high levels.
.png)
Patterns of Time Series: Trend, Seasonality, and Recency Effects
One of aiCast’s key strengths is its ability to decompose time series data into trend, seasonality, and recency effects. This allows the model to understand and capture underlying patterns, making it easier to predict future outcomes. For example, understanding seasonal fluctuations in demand enables better inventory planning, while recency effects help businesses respond quickly to recent shifts in consumer behavior.
.png)
Quantifying and Managing Uncertainty
Uncertainty in business is inevitable. aiCast goes beyond traditional forecasting by not only predicting future outcomes but also quantifying the uncertainty associated with those predictions. This capability is crucial for businesses that need to make informed decisions in stable and unpredictable environments.
- Confidence Intervals: aiCast provides confidence intervals for its forecasts, offering a range of possible outcomes rather than a single point estimate. This allows businesses to prepare for various scenarios and choose the best course of action based on their risk tolerance.
- Scenario Analysis: aiCast supports scenario analysis, enabling businesses to explore different "what-if" scenarios and understand the potential impact of various decisions. This feature is particularly valuable for strategic planning and risk management.
- Actionable Forecasting: aiCast focuses on generating forecasts that are optimized for the specific needs of the business, rather than simply striving for the highest statistical accuracy. The model can account for unique goals such as sustainability, commitments like long-term contracts and other constraints or policies such as safety margins, ensuring that the forecasts are not just accurate, but compliant.
Integration with Historical and Real-time Data for Continuous Learning and Adaptation
aiCast seamlessly integrates both historical and real-time data and is designed to continuously learn and adapt as new data becomes available, ensuring that forecasts are updated to reflect the latest information. This capability is essential for industries where conditions can change rapidly, such as retail, finance, or manufacturing. By combining historical data with real-time inputs, aiCast ensures that its forecasts are not only accurate but also relevant in dynamic environments such as retail demand sensing or predictive maintenance.
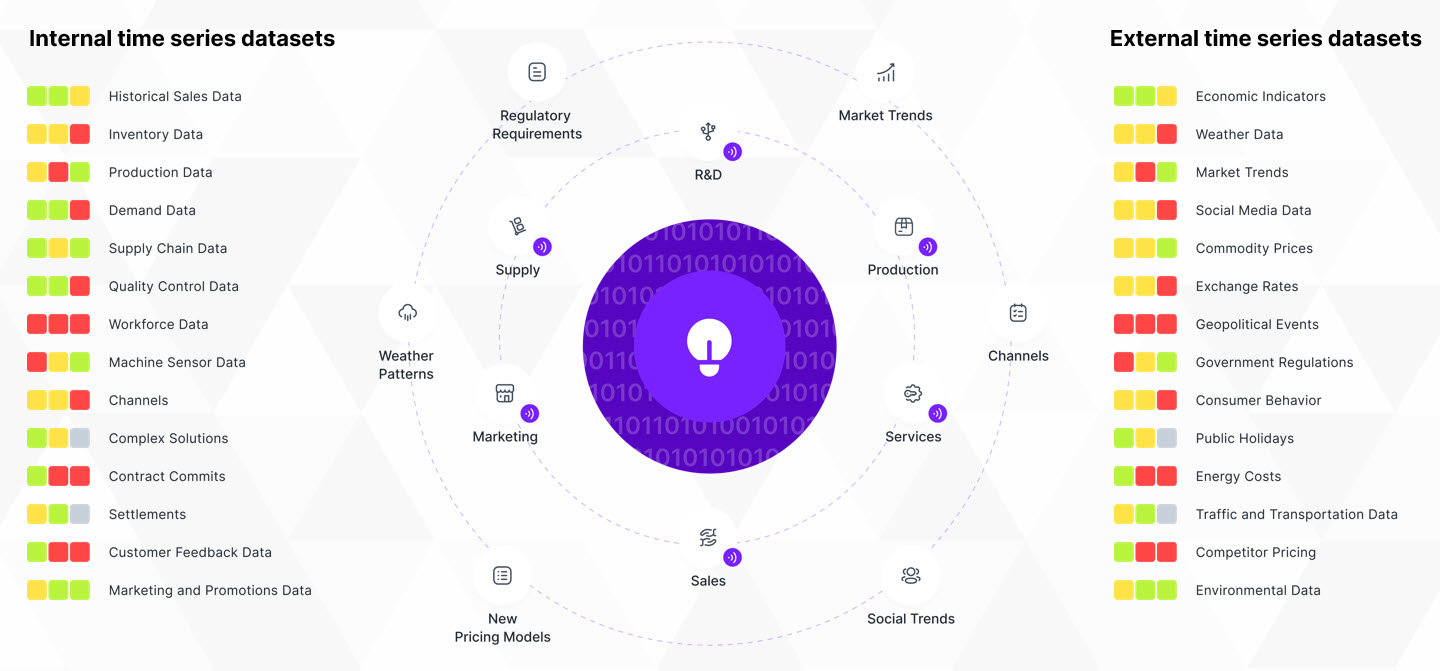
Business benefits: Use cases

Retail Demand Forecasting: Optimizing New Product Launches
A major seasonal retailer uses aiCast to forecast demand for newly launched products every holiday season, even with limited or no historical data. aiCast applied Time2Vec and cohort identification to find similar products, generating forecasts that significantly reduced inventory levels and increased stock availability, ensuring smoother performance during peak season.
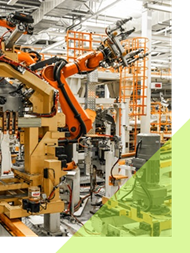
Predictive Maintenance in Manufacturing: Reducing Downtime
In manufacturing, downtime caused by equipment failures can be costly. aiCast uses anomaly detection to identify early signs of potential failures and schedule maintenance before breakdowns occur. For example, by identifying a cohort of machine parts that show similar wear patterns, aiCast enables manufacturing companies to reduce downtime and lower maintenance costs.

Labor Planning Challenges and Disruptions: Managing Change Points
A global apparel and accessories company with a focus on same day delivery faced significant uncertainty in labor planning and shift management due to fluctuating demand and external disruptions especially during promotional periods and seasonal fluctuations. aiCast identified change points in the supply chain—such as transportation capacity and shifts in consumer ordering patterns—and used this insight to adjust forecasts closer to real-time. This capability allowed the company to plan staffing and maintain service levels while minimizing costs, even in volatile market conditions.
aiCast workflow
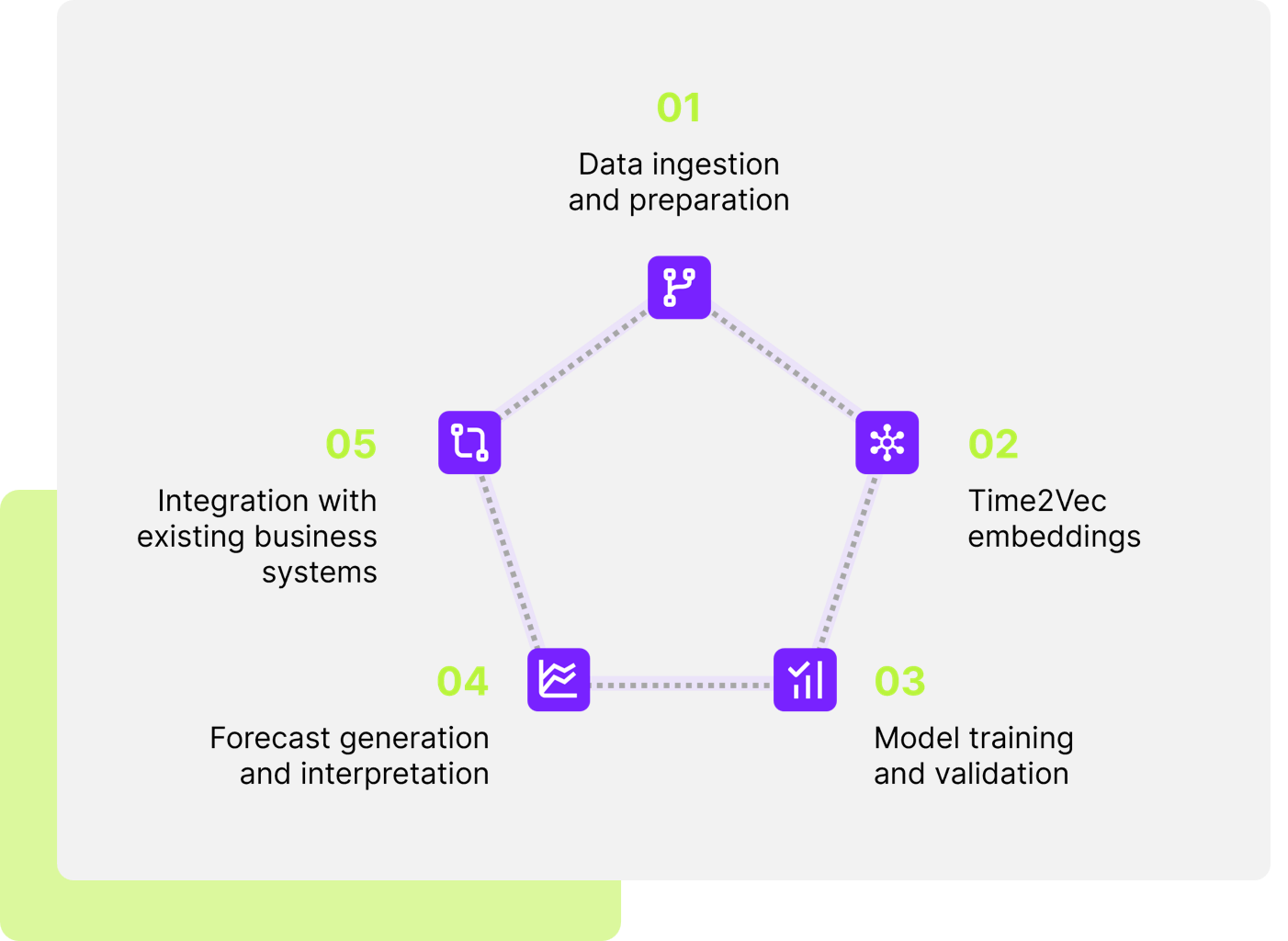
1. Data Ingestion and Preparation
The aiCast workflow begins with data ingestion and preparation, where data from diverse sources (e.g. transactional, operational and external data) are processed to ensure integrity and quality. Thanks to the seamless integration with aiMatch, the resulting clean and normalized data ensures accurate Time2Vec embeddings and generation of insights and forecast based on reliable and complete data sets as well as operational efficiency.
2. Time2Vec Embeddings
Time2Vec is a critical component of aiCast, transforming time series data into structured embeddings that retain key temporal relationships. These embeddings allow aiCast to make accurate forecasts even when historical data is limited, by ensuring that the model understands the underlying patterns and relationships within the data.
3. Model Training and Validation
aiCast employs a range of algorithms to assess different aspects of the input data, pick and run the right techniques and models, validating them against a portion of the data to ensure accuracy. This process includes functionalities such as anomaly detection, cohort analysis, hierarchical reconciliation all managed by autoML. This ensures reliability and relevance of the applied models and the end forecast.
4. Forecast Generation and Interpretation
Once trained, aiCast generates forecasts that include confidence intervals, providing a range of possible outcomes and helping businesses prepare for different scenarios. By incorporating insights from hierarchical reconciliation, aiCast ensures that forecasts remain consistent across different time scales, product and organizational levels and by considering the relationships between different variables, aiCast ensures that these forecasts are both accurate and actionable.
5. Integration with Existing Business Systems
aiCast is designed to integrate seamlessly with existing business systems, ensuring that forecasts are both accessible and actionable. Through API-based integration or a library of standard connectors, aiCast can connect with ERP systems, CRM platforms, and other enterprise tools, enabling businesses to incorporate insights directly into their operational processes.
Expert-in-the-Loop (Xitl) Integration
While aiCast is highly automated, it also incorporates expert feedback through its Expert-in-the-Loop (XitL)feature. For example, in the manufacturing or healthcare sector, experts may provide insights on anomalous market conditions—such as sudden economic shifts, long term contract changes or regulatory requirements —that the model might not fully capture.
By allowing experts to adjust forecasts based on nuanced knowledge, aiCast ensures that the predictions are not only data-driven but also aligned with real-world experience and business knowledge.
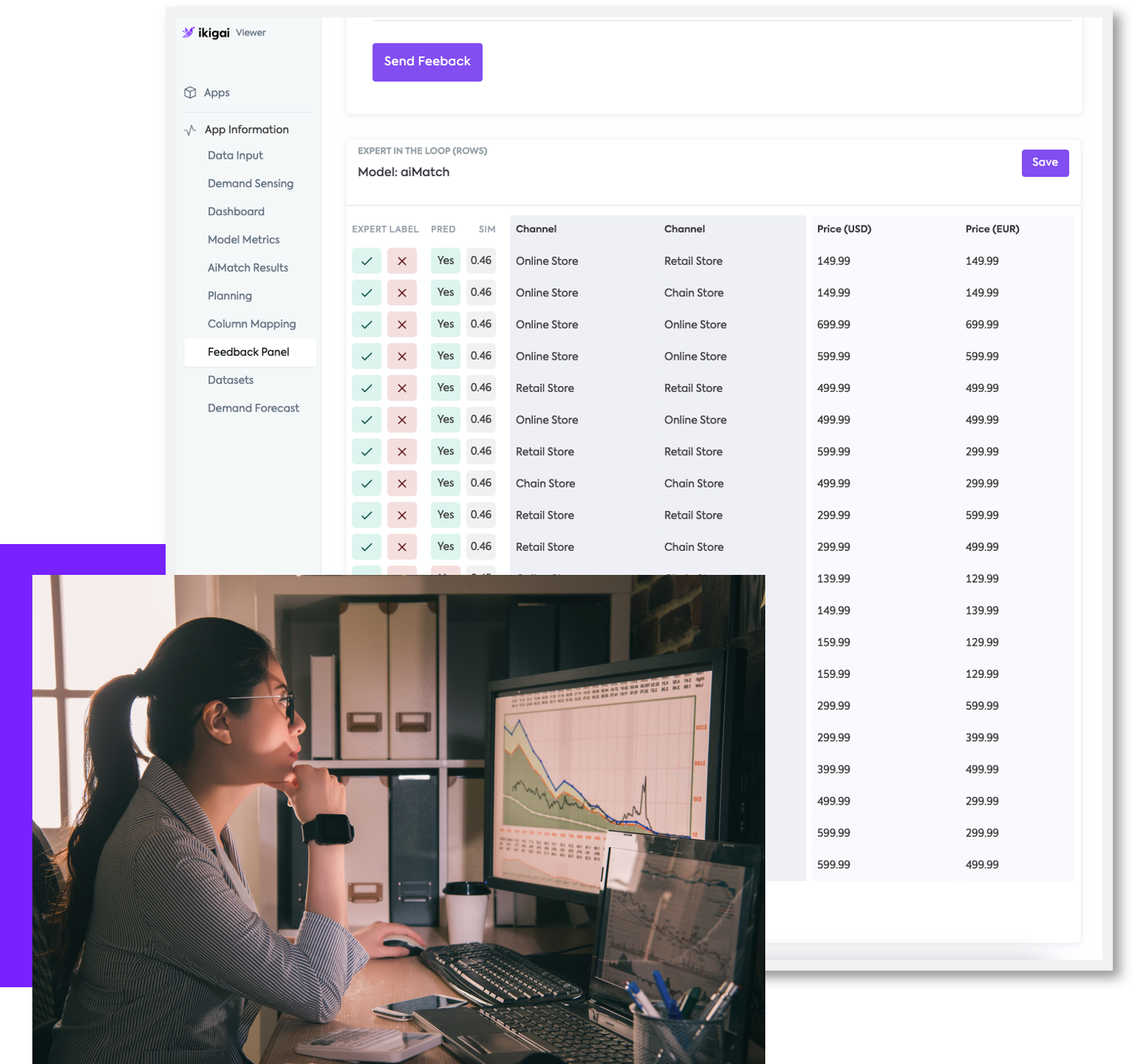
Conclusion
aiCast represents a significant advancement in forecasting technology, offering businesses a tool that not only provides accurate predictions but also integrates expert feedback, handles missing data, and adapts to changes in time series dynamics. As businesses continue to face rapidly changing markets and increasing complexity, the ability to forecast with precision and adaptability will be key to sustaining competitive advantage. Whether launching a new product, managing supply chain disruptions, or optimizing financial strategies, aiCast’s ability to deliver tailored, actionable forecasts makes it a critical AI capability for business.
Additional resources
Glossary
aiCast
aiCast is a forecasting AI model based on patented Large Graphical Models (LGM). It is designed to predict future trends and outcomes based on both historical tabular and time series data and real-time data. aiCast generates 20% more accurate forecasts than traditional models and methods, even with sparse data.
aiMatch
aiMatch is a data reconciliation AI model based on patented Large Graphical Models (LGM). It automates the process of connecting and harmonizing disparate datasets, ensuring consistency and accuracy across multiple sources. By utilizing advanced pattern recognition and probabilistic techniques, aiMatch enables identification and resolution of inconsistent data and can synthesize new data to address missing or incorrect data.
aiPlan
aiPlan is a scenario planning AI model based on Large Graphical Models (LGM) which can generate and evaluate up to 1019 scenarios based on complex datasets. By simulating various potential outcomes and their likelihoods, aiPlan enhances scenario planning by providing insights into risks, opportunities, and strategic responses for organizations to navigate uncertainties.
Cohort analysis
Grouping similar time series together for targeted forecasting, ensuring that forecasts are relevant to specific business contexts.
Confidence interval
A range of values derived from a forecast, indicating the degree of uncertainty around the predicted outcome.
eXpert-in-the-Loop
"eXpert-in-the-loop" (Xitl) refers to a hybrid approach in artificial intelligence where human expertise is integrated into the machine learning process. This methodology involves combining the capabilities of machine learning algorithms with human domain knowledge or judgment to improve the accuracy, efficiency, and interpretability of AI systems.
Hierarchical Reconciliation
Ensuring consistency across various levels of data aggregation, making forecasts accurate at both granular and high levels.
Large Graphical Model (LGM)
A Large Graphical Model is a generative AI model that produces a graph to represent the conditional dependencies between a set of random variables. It is designed to work with enterprise-specific or proprietary data sources, such as tabular and time series data used in data reconciliation, forecasting, and scenario planning.
Quantifying Uncertainty
The process of measuring and expressing the uncertainty in a forecast, typically through confidence intervals or scenario analysis, to aid in decision-making.
Download PDF
To download the eBook as a PDF, click here.
To learn more about the Ikigai platform, visit here.