Unlocking the Power of aiPlan:
Evaluating Tradeoffs for Optimal Business Results
Last update: April 26, 2024
Introduction
In an increasingly complex and uncertain business environment, traditional forecasting methods often fall short in providing insights for decision-making. AI-powered scenario planning, on the other hand, offers a more structured and strategic approach to understanding and managing uncertainty, allowing organizations to identify potential risks and opportunities, assess their impact on key constraints, and develop data-driven strategies to meet business goals.
By analyzing various future states and assessing their potential implications, scenario planning empowers organizations to make more informed decisions, adapt to evolving circumstances, and thrive in uncertain times.
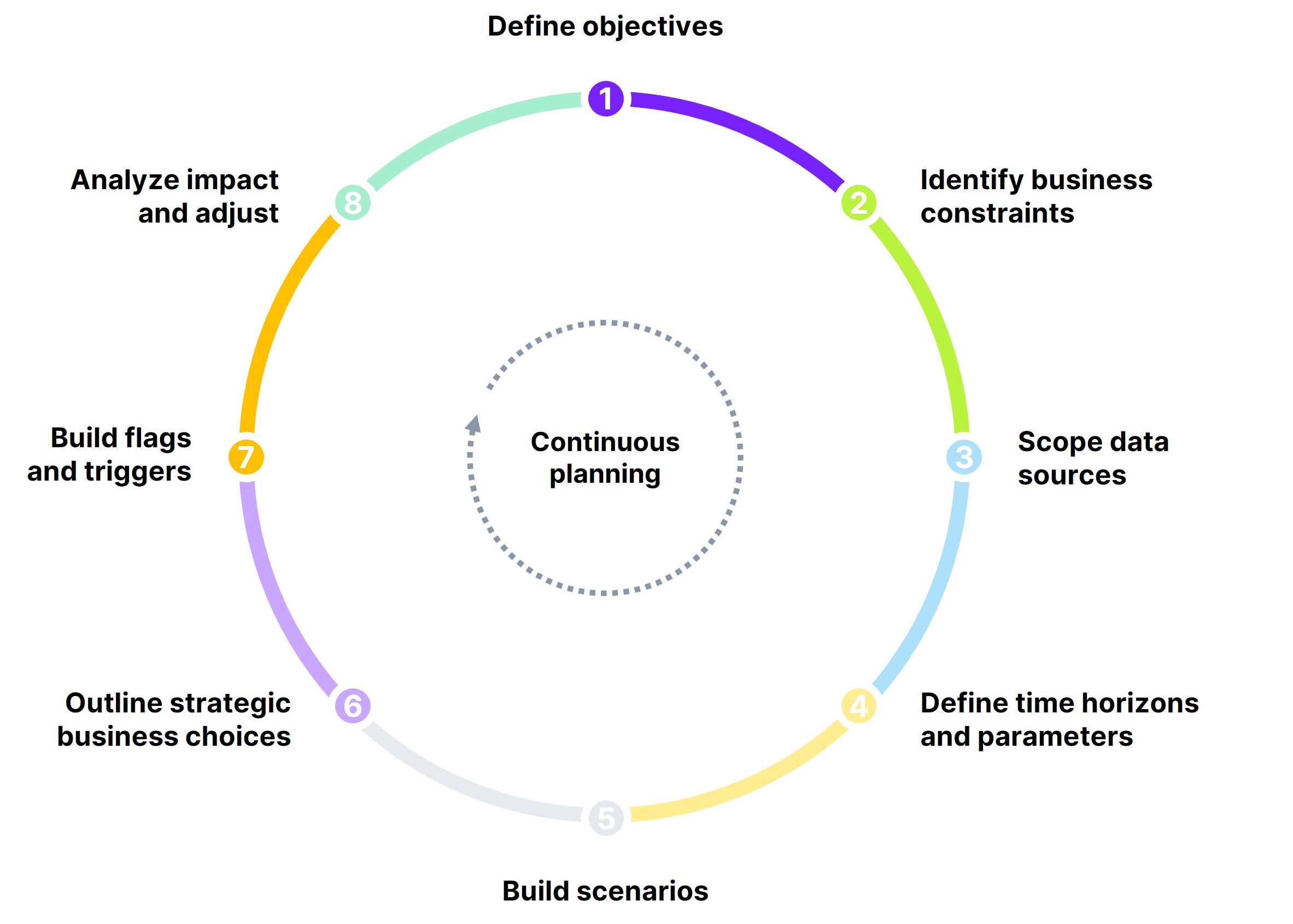
In this eBook, we will explore scenario planning within the context of the supply chain, where tradeoffs between supply and demand have significant financial and reputational impact. We’ll then look at novel approaches to this problem based upon Large Graphical Models, designed specifically for forecasting and modeling tabular and time series data.
Use LGMs to evaluate options and tradeoffs
Ikigai is a Generative AI platform that transforms how businesses handle internal and external structured data, particularly timestamped information, turning it into actionable insights. Grounded in award-winning MIT research, Ikigai’s patented Large Graphical Model (LGM) powers the platform’s aiMatch, aiCast, and aiPlan models and industry solutions. These enable tabular and time series data reconciliation, forecasting, scenario planning, and outcome optimization.
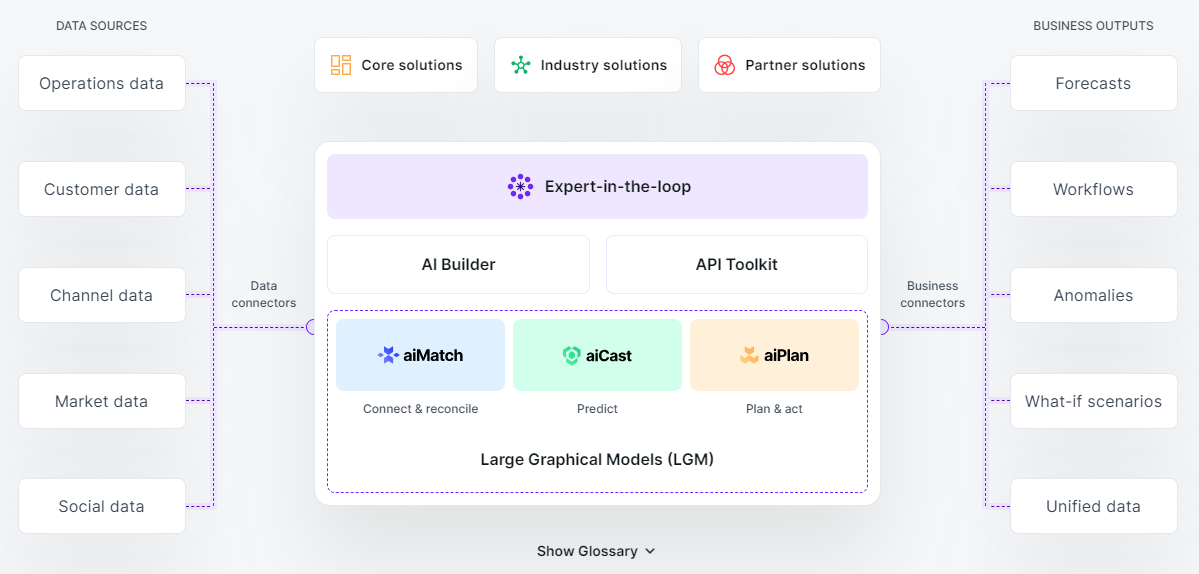
With aiPlan from Ikigai, organizations can automatically generate and evaluate thousands of different scenarios, incorporating both internal and external data to produce more accurate business insights and recommend-dations. And compared to traditional methods of scenario planning which are primarily based on static rule sets, aiPlan continuously iterates, incorporating new information and business priorities to deliver greater agility and resiliency.
With aiPlan, organizations can automatically generate and evaluate thousands of different scenarios to produce more accurate business insights and recommendations.
Scenario Planning
Balancing supply and demand across the supply chain
The primary goal of the supply chain is to balance supply and demand as accurately as possible in a just-in-time manner. Operating a supply chain amidst complexity and uncertainty, coupled with unpredictable shifts in demand and limited capacity to influence it, is highly challenging.
At extremes, one can plan for very little supply and not ever risk over stocking or plan for too much supply and not ever risk lost demand. Both have fatal consequences for the business. Therefore, it is essential to understand all the plausible tradeoffs achievable between various competing outcomes of interests (including cost of operating supply chain and risk of unfulfilled demand).
Scenario planning precisely enables this understanding in quantitative manner by providing a pareto boundary of tradeoffs between various end-outcomes of interests accompanied by policies that can help provide granular recommendations to achieve the chosen tradeoff between end outcomes. The policy continually adapts recommendations to account for changing environments while keeping an eye on the end objective. This is achieved through solving a complex reinforcement learning problem.
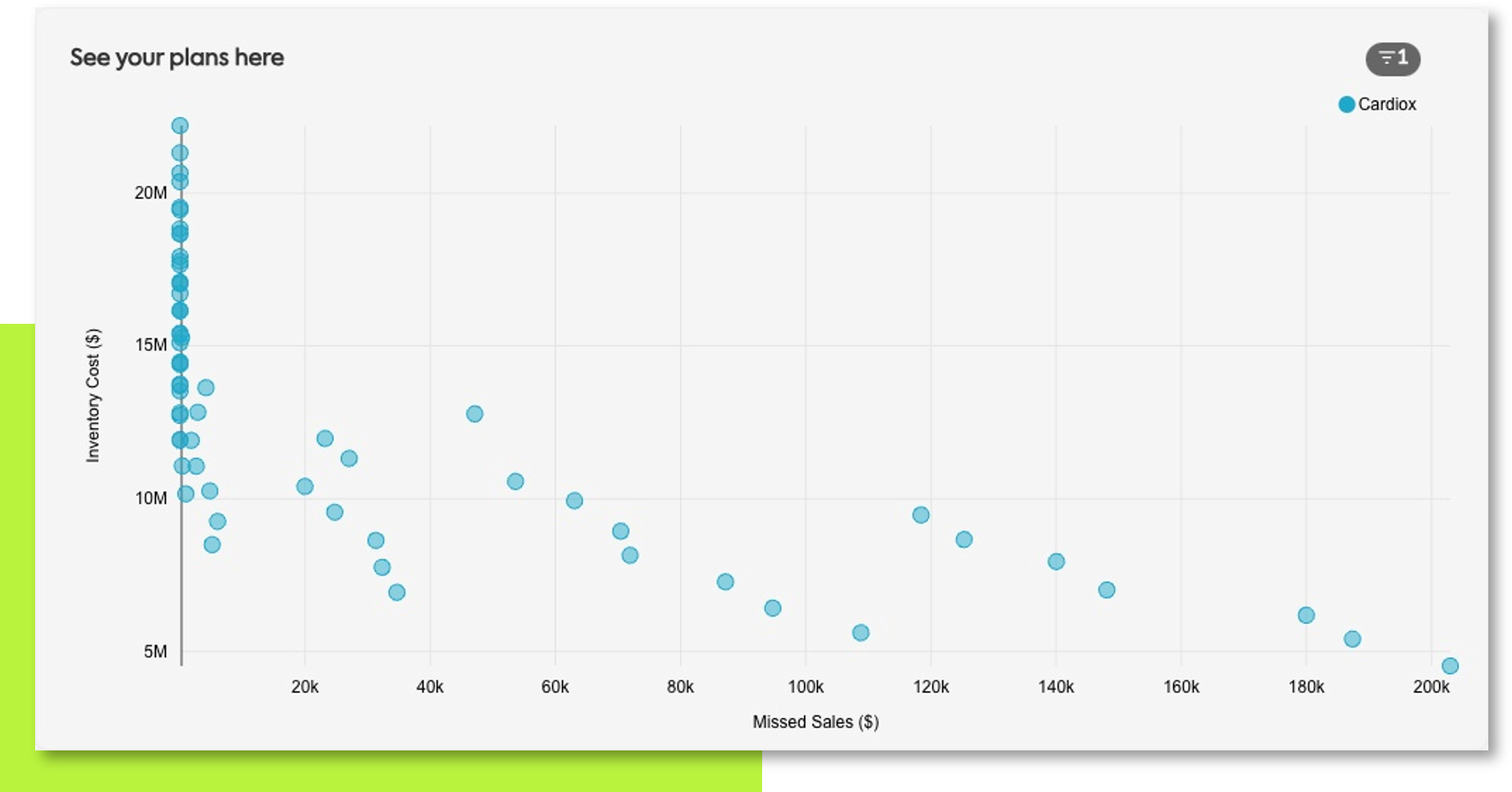
The example here illustrates a pareto boundary of tradeoffs achievable between inventory holding cost and lost demand. Each dot in the diagram corresponds to an achievable tradeoff and corresponds to a policy that can provide granular level operating decisions across supply chain network with aim of achieving this tradeoff over the planning horizon. The policy adapts its recommendations continually in face of changing environment.
For example, it can provide a pareto boundary of feasible tradeoffs between cost of inventory (overstocking) and missed sales (risk of not fulfilling demand). This could be a multiple objective tradeoff including achievable tradeoffs, for example between overall cost of inventory, missed sales (aka risk of not fulfilling demand) and availability (in-store versus ship by air versus by road or water).
Typically, the selection of the operating point in this tradeoff pareto boundary is done with the overall strategic and operating outcomes and goals in mind. This may be done at a lower frequency or higher frequency.
Pareto boundaries define the boundary between feasible and unattainable outcomes in a multi-objective optimization problem, illustrating the tradeoffs between competing objectives
Recommendation Actions
The selection of an operating point (or policy) involves choosing between a specific combination of tradeoffs between different objectives or goals, for example cost, service level, and inventory levels, that the supply chain aims to achieve. The chosen operating point then leads to specific operating recommendations or guidelines on how to run the supply chain operations to realize the targeted tradeoff. In this example, the output may provide recommended inventory levels, production quantities, reserved transportation capacity, etc. These recommendations are integrated with information about the supply chain network such as capacity, costs, lead times, ensuring that recommendations respect real-world operational constraints.

The recommendations continuously adapt as the business environment changes (demand, supply, costs, etc.), dynamically adjusting to maintain desired balance or tradeoffs between the key objectives coming out of the scenario analysis.
Additionally supply chain experts can manually edit (expert-in-the-loop) or override certain recommended actions based on their knowledge Such edits change the ‘state’ or configuration of the supply chain system, which would trigger updates or other recommended actions to maintain achievement of the objective. For example, if the recommended action at an intermediate stage of the supply chain is “edited”, to make it consistent for upstream and downstream stages, to the extent possible, the recommended actions are updated for each stage.
In summary, aiPlan facilitates a responsive, iterative process for selecting and adjusting strategic tradeoff objectives and generating detailed operational recommendations to achieve those objectives within business or operational constraints while allowing expert input to shape the recommendations.
Integration with aiCast
To develop effective scenario plans and policy recommendations, we need to model the uncertainties that can impact operations. This includes forecasting demand as well as accounting for uncertainties in external value chain or market factors like transportation, manufacturing, and 3rd parties like suppliers of suppliers.
.png)
aiCast utilizes historical and forecasted data to develop and run simulations that model potential business scenarios. This allows the model to identify the optimal tradeoffs and associated operational policies to achieve the desired business objectives at any level of granularity including day-to-day or week-to-week.
In that sense, aiCast (forecasting) is naturally integrated into an effective, continuous planning process capable of integrating runtime information, rather than simply being an event-based input to an (eventual) end.
Network Model
Incorporating existing supply chain network constraints
The supply chain network has multiple interconnected stages – sourcing raw materials from vendors, manufacturing at plants with capacity constraints, transportation, distribution centers and other intermediary steps. This multi-stage structure creates inter-dependencies that must be accounted for in the planning process to properly account for uncertainty at each stage along with the overarching constraints.
The aiPlan module models these granular network constraints to build a system dynamics model. Combined with aiCast (which leverages historical data), this enables generating realistic simulations and subsequently deriving operational policies that provide recommended actions that are consistent across the network or system.
Incorporating the supply chain network model allows for accurate scenario planning by explicitly considering both the constraints and the uncertainties inherent to the network. Each policy derived by aiPlan corresponds to the chosen tradeoff between the key outcomes or objectives of interest. It prescribes granular decisions at the appropriate level and granular time scale such as which vendor to order materials from, quantities, timing, transportation mode for different sales end points, etc.
Tradeoffs with Sustainability

The modes of shipping for product distribution play an important role in determining the impact of supply chain on the environment. However, modes of shipping come with different characteristics, for example, shipping by sea takes a lot longer compared to shipping by air, and transporting through certain ocean routes can be less predictable.
aiPlan can incorporate these as part of the modeling of the supply chain stages and options. Using aiCast (with historical data), it can model the uncertainty in the transportation time and cost. Collectively they provide a holistic simulation model to enable scenario planning between sustainability, operating cost, and potential of lost demand.
The pareto boundary of such tradeoffs enables decision makers to choose the operating point which then translates into recommended decisions at a granular level.
Cost Efficient Planning
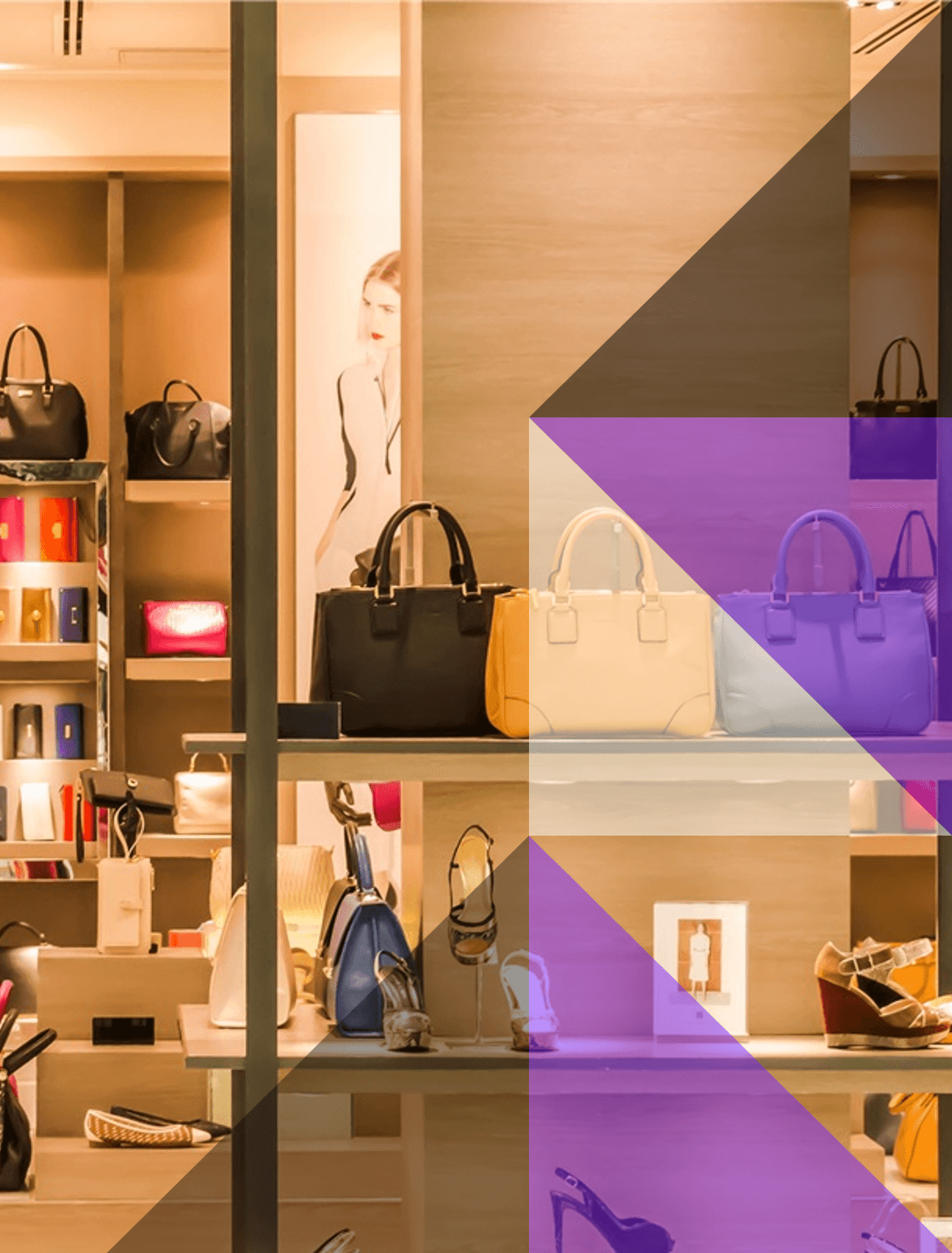
The tradeoff between cost of operating supply chain and the risk of unfulfilled demand is at the core of supply chain operations. aiPlan and aiCast together enable identifying the pareto boundary of the tradeoffs between these objectives. It will enable comparison with the existing operating point and provides immediate understanding of plausible improvements achievable.
For example, a large seasonal retailer found that by using aiPlan scenario analysis, they could reduce their inventory holding cost by close to 50% and still fulfill the same demand. Such cost savings can be applied across range of product lines with different planning frequency.
Conclusion
AI-powered scenario planning is a strategic differentiator in today’s dynamic business landscape. And in the complex and often volatile world of supply chain where disruptions can cost companies an average of 40% of their annual earnings, leveraging AI-based scenario planning enables businesses to identify potential risks and proactively respond to changing circumstances.
The combined power of Ikigai’s aiCast and aiPlan models deliver significantly greater forecast and planning accuracy based on patented Large Graphical Models designed for tabular and timeseries data. Going beyond traditional rules-based forecasting and planning methods, Ikigai incorporates vast amounts of historical and external data to generate thousands of scenarios and assess their potential impact on a business’ supply chain operations. This enables supply chain professionals to identify potential risks, optimize resource allocation, and enhance resiliency.
Additional resources
- aiMatch data reconciliation
- aiCast forecasting
- aiPlan scenario plannng
- How to transform your business outcomes with generative AI
- The case for probabilistic versus deterministic forecasting and planning
Glossary
aiCast
aiCast is a forecasting AI model based onpatented Large Graphical Models (LGM). It is designed to predict future trendsand outcomes based on both historical tabular and time series data andreal-time data. aiCast generates 20% more accurate forecasts than traditionalmodels and methods, even with sparse data.
aiMatch
aiMatch is a data reconciliation AI model based on patented Large Graphical Models (LGM). It automates the process of connecting and harmonizing disparate datasets, ensuring consistency and accuracy across multiple sources. By utilizing advanced pattern recognition and probabilistic techniques, aiMatch enables identification and resolution of inconsistent data and can synthesize new data to address missing or incorrect data.
aiPlan
aiPlan is a scenario planning AI model based on Large Graphical Models (LGM) which can generate and evaluate up to 1019 scenarios based on complex datasets. By simulating various potential outcomes and their likelihoods, aiPlan enhances scenario planning by providing insights into risks, opportunities, and strategic responses for organizations to navigate uncertainties.
eXpert-in-the-Loop
"eXpert-in-the-loop" (Xitl) refers to a hybrid approach in artificial intelligence where human expertise is integrated into the machine learning process. This methodology involves combining the capabilities of machine learning algorithms with human domain knowledge or judgment to improve the accuracy, efficiency, and interpretability of AI systems.
Large Graphical Model (LGM)
A Large Graphical Model is a generative AI model that produces a graph to represent the conditional dependencies between a set of random variables. It is designed to work with enterprise-specific or proprietary data sources, such as tabular and time series data used in data reconciliation, forecasting, and scenario planning.
Operating Point
The operating point represents the strategic position an organization chooses to adopt within a given scenario. It reflects the decisions and actions taken to navigate uncertainties and capitalize on opportunities presented by the envisioned future conditions.
Pareto Boundary
Pareto boundaries define the boundary between feasible and unattainable outcomes in a multi-objective optimization problem, illustrating the trade-offs between competing objectives. They encapsulate the Pareto optimal solutions, where no further improvement in one objective is possible without sacrificing another.
To learn more about the Ikigai platform, visit here.
To download the eBook as a PDF, click here.