The Case for Probabilistic vs Deterministic Forecasting and Planning
Enabling certainty (business outcomes) in an uncertain environment
Last update: April 4, 2024
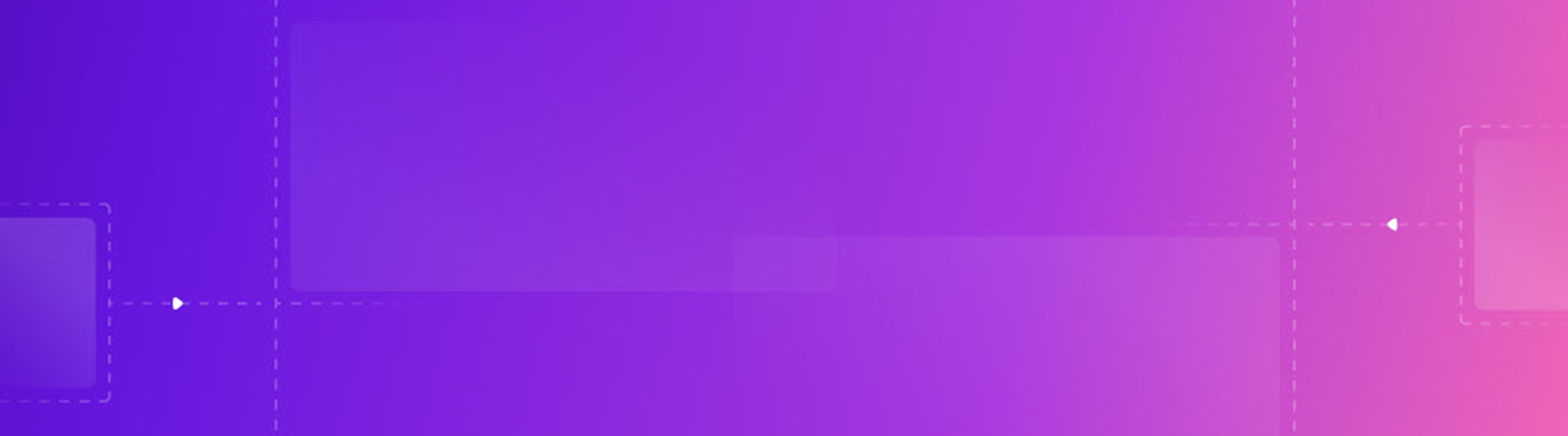
Overview
In today's dynamic and uncertain business environment, traditional deterministic forecasting and planning methods can’t keep up with rapid variability and changes in demand and supply patterns. Three immediately pertinent examples include:
- Global socio-economic and supply chain disruptions
- Fluctuations in raw material availability reliant on a dispersed supplier base
- Rapidly evolving and influenced consumer preferences
These challenges create significant stress for those responsible for forecasting and planning, as traditional methods are rigid by design, relying on pre-determined - and often outdated - rules to drive decision-making. In this article I delve into added advantage of incorporating probabilistic forecasting and planning particularly in environments marked by external influences, uncertainty or volatility, contrasting it with exclusively deterministic approaches commonly found in solutions like O9 and SAP IBP. While these examples focus on the manufacturing vertical, the general principles apply to any business environment that is inherently uncertain. It is important to note that the choice between deterministic and probabilistic approaches is not binary.
It is important to note that the choice between deterministic and probabilistic approaches is not binary.
The most effective solution in some cases may be to use both methods in tandem with probabilistic frameworks thus creating the added advantage. This allows businesses to practically leverage the respective strengths of the two approaches based on the specific use case and forecasting or planning horizon.
Deterministic Forecasting Explained
Deterministic forecasting is a method of prediction that tries to predict a single, "best-case" value for future demand, assuming future outcomes can be precisely determined based on known variables and mathematical models. Relying on historical data and statistical techniques to identify patterns and trends, deterministic forecasting works well in situations where predictable patterns can be observed, and where small variations don't have major (cost) implications.
Some common methodologies used in deterministic forecasting include:
- Moving Averages: This method calculates the average demand over a defined period (e.g., weekly, monthly) and projects this average into the future. It's effective for capturing seasonal trends but can struggle if there are sudden changes in demand patterns.
- Exponential Smoothing: This method assigns weights to past data points, with more recent data points receiving higher weights. This allows the forecast to adapt to recent trends but may not capture long-term seasonality as effectively as moving averages.
- Regression Analysis: This technique identifies statistical relationships between demand and influencing factors (e.g., price, marketing campaigns). A mathematical model is then built to forecast future demand based on these relationships.
Deterministic forecasting is best suited for stable or predictable environments, short-term operational forecasts, and compliance-driven industries. However, it struggles with uncertainty and change and may miss important risks and opportunities.
Example: Deterministic Forecasting in an IBP platform (Scenario: Electronics demand)
Imagine an electronics manufacturer using an IBP solution for deterministic forecasting. In this scenario, the company enjoys a largely stable market with high barriers to entry because of a lock in with regulators as sole source provider. To predict next quarter’s demand, they might use a combination of moving averages and exponential smoothing to analyze past sales data of a specific product model and consider factors like seasonal trends and the outcomes of past marketing campaigns. Based on this analysis, the forecasting solution might predict a deterministic demand of 10,000 units for the next quarter using a weighted average of past sales figures.
Limitations of Deterministic Forecasting in a Highly Variable Business Environment
The success of the previous example depends largely on relatively high levels of business stability. Since most organizations today operate in an inherently unstable environment, the following conditions subvert the effectiveness of deterministic models:
- Business model or environment shifts: Deterministic models typically lack flexibility to adapt to significant or unexpected changes in data distribution or market dynamics (e.g., new product categories, new supplier channels or supplier type (e.g., marketplaces) or regional shifts). This hinders their effectiveness in a constantly changing environment.
- Rapid uncertainty in demand and supply: The post-Covid world has seen higher levels of systemic variability in demand and supply, along with inflation and socio-economic challenges that exacerbate the instability of the economic environment. Lead times, material availability, and customer behavior have become more unpredictable, and in some cases a pendulum of action and reaction has followed (overinvestments in capacity, lead time reduction, near-shoring, vertical integration while customer demand has dissipated). Deterministic forecasting is not suited to handle the inherent variability in demand, supply chains, and other critical factors, and struggles to assess the risks associated with different scenarios, leading to poor resource allocation, and missed opportunities when planning,
In a world of rapidly changing conditions, relying on a single point estimate or even range for forecasting creates a false sense of certainty. If actual demand deviates significantly (either higher or lower) than the predicted outcomes, businesses are unprepared to capitalize on opportunities or mitigate potential stockouts, leading to lost sales or alternatively unnecessary inventory carrying costs.
In a world of rapidly changing conditions, relying on a single point estimate or even range for forecasting creates a false sense of certainty.
When sole reliance is placed on a single point estimate, businesses are forced to take a reactive approach when things don’t go as planned, resulting in costly scrambles to adjust production or inventory levels when demand fluctuates unexpectedly.
Probabilistic Forecasting Explained
Probabilistic forecasting acknowledges the inherent uncertainty in demand by generating a range of potential outcomes with their associated probabilities. In high-variability environments, the approach creates a more realistic picture, allowing for better decision-making while empowering proactive planning within the range of certainty or uncertainty posited by the forecast. Some common methodologies used in probabilistic forecasting include:
- Monte Carlo Simulation: This method simulates many possible scenarios by randomly sampling from historical data and incorporating variability factors. The resulting distribution of outcomes provides a probabilistic forecast.
- Bayesian Networks: These graphical models represent relationships between variables that influence demand. By updating the network with new data, Bayesian networks can generate probabilistic forecasts that reflect the changing dynamics of the market.
Probabilistic forecasting is best suited for dynamic and uncertain environments, medium- to long-term strategic forecasts, and data-rich industries. However, it can require more data and technical expertise and must be weighed in the context of the use case value.
Example: Probabilistic Forecasting (Scenario: Medical Device)
A medical device company focused on asthma care can leverage probabilistic forecasting to plan for the demand of its infusion therapy treatment. The company may analyze historical sales data, as well as external inputs such as weather forecasts, temperature, humidity, reimbursement levels, and competitor promotions. In a probabilistic model, predictive variables aggregate these influencing factors at appropriate levels of dimensionality.
In a probabilistic model, predictive variables aggregate these influencing factors at appropriate levels of dimensionality.
Based on this analysis, it might generate a probabilistic forecast indicating a 60% chance that between 50,000 and 70,000 infusion therapy procedures will happen onsite, and 150,000,000 administrations will be home-based in the next quarter, with a 20% chance of demand exceeding 70,000 onsite uses, and a 20% chance of demand falling below 50,000 uses.
The Power of Probabilistic Forecasting:
In the previous example, the success of the probabilistic planning approach hinged on its ability to adapt to the variability of allergy season, including unexpected weather fluctuations and more. Unlike deterministic models, which falter in such dynamic settings, the following strengths make probabilistic approaches ideal for navigating the instability of the modern business landscape:
- Modeling for variability or a range of values: Instead of a single point estimate, the forecast considers systematic variability, such as a range of potential lead times (e.g., 8-16 days) for refills, and other variable parameters (e.g., insurance coverage limits.) This range is then translated into probabilities, providing a more comprehensive picture and ability to make informed decisions about potential outcomes.
When organizations can de-risk planning in an intrinsically variable world, results and benefits are manifold. They include:
- Increased forecast accuracy: By acknowledging variability, forecasts are more likely to reflect real-world demand patterns, leading to improved prediction accuracy. Let’s illustrate this benefit by considering the alternative. With deterministic forecasting and planning, if actual results fall outside the anticipated “range” of outcomes, (i.e., something unexpected happens) you are in an unknown setting and risk a serious downstream impact. In the case of demand planning, an unexpected event can quickly result in loss of business and goodwill.
On the other hand, probabilistic forecasting anticipates a range of possibilities while probabilistic planning enables a range of contingency plans. This tight interlock of forecasting and variability across any possible set of outcomes and constraints is the foundation for operating excellence in an uncertain environment. - Enhanced scenario planning: Exploring "what-if" scenarios is a powerful capability enabled by probability. Building on the medical device example, one could use probabilistic forecasting to simulate the potential impact of an unexpected increase in insurance coverage campaign by Medicaid. Based on this analysis, the business could proactively develop contingency plans to address different scenarios and make proactive adjustments to inventory levels and marketing strategies. This is a core aspect of business continuity planning in the face of unexpected events.
- Improved resource management: Probabilistic forecasts enable businesses to determine all feasible business risk vs reward scenarios for a given set of outcomes and constraints. For example, a manufacturer may make larger investments in inventory to reduce the risk of stock outs, but these additional investments increase the risk of excessive holding costs. By understanding the plausible tradeoffs in play, probabilistic planning supports data-driven inventory management decisions. In the previous example, a clear understanding of the probability of different demand levels would allow a retailer to set optimal safety stock levels that minimize the risk of stockouts while avoiding excessive inventory holding costs.
...probabilistic forecasting anticipates a range of possibilities while probabilistic planning enables a range of contingency plans.
Additional benefits specific to a demand planning and management context include:
- Reduced inventory holding costs because of inventory planning that is driven by demand signals.
- Reduced stockout costs due to better anticipation of demand fluctuations.
- Improved customer satisfaction resulting from reduced wait times and increased product availability.
- Potential increase in market share from the ability to meet customer demand consistently versus competition.
Operating in an Era of Uncertainty: Modern Supply Chain Considerations
The improved accuracy, stability, demand sensitivity and resilience of probabilistic approaches takes on particular importance in uncertain supply chains along four key dimensions as explained below.
- Transactional Frequency: Unlike deterministic systems that rely largely on historical averages or expert intervention to incorporate ongoing changes, probabilistic systems account for daily, weekly, or even hourly variations in demand by incorporating probability distributions to deliver predictions to the highest desired level of business granularity.
- Forecasting and Planning Frequency: Deterministic systems may require frequent updates due to above mentioned limitations, making administrative effort and cost a serious issue, as well as challenges related to versioning, reproducibility, and audibility with differing versions of rules and data. Probabilistic systems are built to learn from the data and reflect real time changes in its view of future demand.
- Hierarchical planning: Deterministic systems are challenged with reconciling uncertainties across various levels for the dimensions that drive business including product, supplier, geo, channel, or book of business. Probabilistic models are based on the propagation of uncertainty through different levels of hierarchy. This enables both a top-down and bottoms-up approach to planning while also building confidence for business owners managing SLAs at each level.
- Uncertainty: Deterministic systems ignore dynamics such as changing customer preferences or competitor actions. In contrast, probabilistic systems explicitly learn and implement learned distributions for these variables within a range of confidence. This enables proactive planning for various possibilities.
Not one or the Other: The Power of Complementary Approaches
In many cases, the most effective solutions may be to use deterministic and probabilistic approaches in tandem, leveraging the strengths of each as appropriate. For example
- Use deterministic approaches for short-term tactical decisions and probabilistic methods for long-term strategic planning
- Leverage probabilistic forecasting and planning for high-impact, high-variability areas and utilize established rules and methods for stable or predictable parts of the business
- Employ probabilistic models to identify key drivers and uncertainties, then use these insights (features) to refine and improve deterministic forecasts.
- One powerful way in which the added advantage of probabilistic forecasting can complement and enhance rules-based systems is through the use of unsupervised, domain-agnostic models. These can identify hidden patterns and relationships in data, adapt to changing conditions while learning from new data over time and quantify the uncertainties that impact businesses.
In conclusion, probabilistic forecasting is a powerful approach that can help businesses navigate uncertainty, make better decisions, and drive real impact. By leveraging the strengths of both probabilistic and deterministic methods in a strategic and targeted way, organizations can create more accurate, adaptive, insightful, and actionable forecasts that drive better outcomes in today's dynamic business environment.
Representative Case Study
No real company data is used
Acme Corp. is a leading manufacturer of home security systems with a flagship product, the Experis. The company has enjoyed a stable market position in the past driven by steady demand for its core products and a loyal customer base. Acme has been using an integrated business planning system based on deterministic forecasting to predict demand for its products.
In this scenario Acme uses a combination of moving averages and exponential smoothing to analyze past sales data of specific home security models, considering factors like seasonal trends, housing market conditions and the outcomes of past marketing campaigns. Based on this analysis, the forecasting solution might predict a deterministic demand of 75,000 units for each of the two next quarters using a weighted average of past sales figures.
However, the home security market is evolving rapidly, with the emergence of new technologies like smart home integration, AI-powered video software solutions and IoT connectivity. Moreover, external factors such as economic conditions, competitive challenges that impact the category, crime rates and consumer preferences can introduce significant variability in demand patterns. Relying solely on deterministic forecasting methods may limit Acme Corp’s ability to navigate uncertainty and adapt to market shifts.
In the case study below, probabilistic forecasting proves to be a valuable addition to Acme’s existing deterministic approach, enabling the company to better navigate growing competitiveness and business uncertainty in the home security products market. While deterministic forecasting remains relevant for certain stable product lines such as basic security sensors or traditional alarm systems with long sales history, by leveraging probabilistic methods, the company can adapt to market disruptions, assess competitive, customer sentiment and economic risks and in turn drive more informed forecasts, assess risks and make appropriate resource and spend decisions.
Before adopting probabilistic forecasting
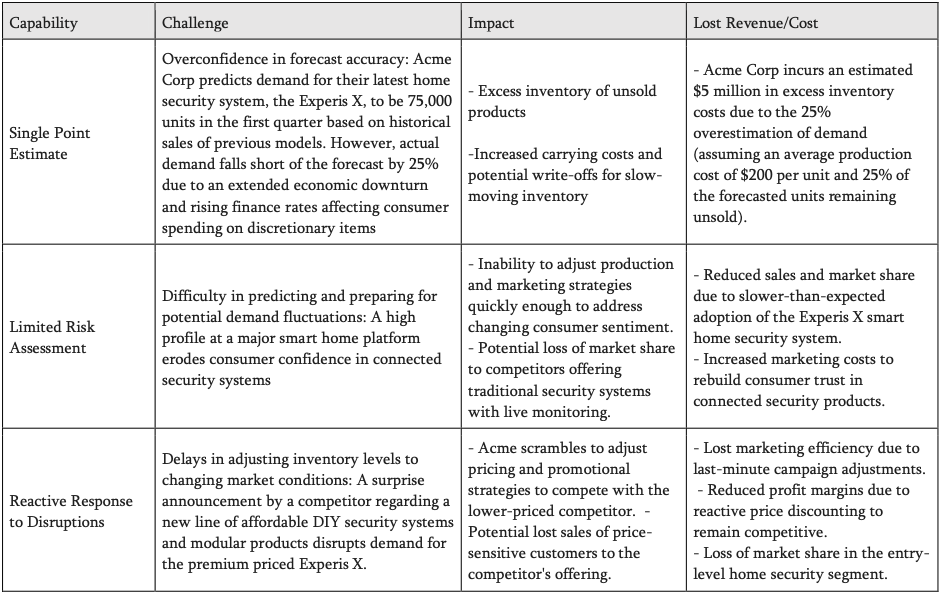
After adopting probabilistic forecasting and planning

Download PDF
Click here to download this content in PDF format.