For Retailers, the Money’s in the Merchandizing
With the advent of AI, retailers can now dynamically surface insights from a wide range of internal and external sources to accurately predict customer demand and test the potential effectiveness of different merchandizing strategies.
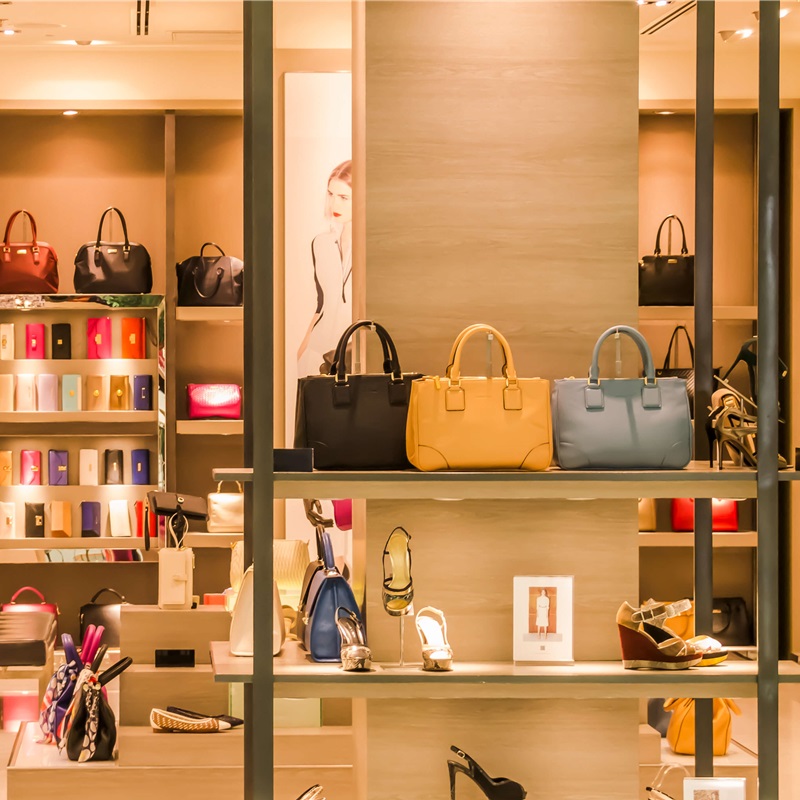
Growing sales through better merchandizing strategies
For retailers, merchandizing is the secret sauce to success. Even a good product can’t sell itself; it relies on a complex range of practices to attract customers and influence their purchasing decisions. For example, retailers must consider pricing strategies, promotional campaigns, product packaging and placement, as well as omnichannel tactics. And all of this is highly influenced by customer demand, market trends, and a host of other external factors that sometimes appear out of nowhere. It’s like a Rubik’s Cube of seemingly endless variables for planners.
Fortunately, merchandizing has a new ally. With the advent of AI, retailers can now dynamically surface insights from a wide range of internal and external sources to accurately predict customer demand and test the potential effectiveness of different merchandizing strategies. One such example is Nike, which collects user data through various Nike apps, then uses AI to identify which designs are the most popular and what products to stock across its e-commerce and retail locations.
AI as a competitive advantage
In our digital world, AI is essential in building an effective merchandizing strategy. Retailers are flooded with data – from sales transactions, social sentiments, apps, and product sensors. Traditional spreadsheets and planning apps aren’t equipped to connect and unify all these disparate sources and translate them into accurate forecasts and plans. And while generative AI technology such as Large Language Models (LLMs) are extremely effective tools in the retail sector for text-based use cases like customer support, personalization, and content generation, they are not designed for the multivariate time series data that feeds retail forecasts and plans.
What’s unique and different about time series data? For one, it’s multidimensional, lending itself to vast combinations of relationships that can surface new insights and strategies. Does a particular product perform better when placed next to another? How do certain promotional campaigns influence customer demand? Which SKUs perform better online versus in-store, and why? How will inflation concerns impact buying behavior?
Unlocking the value of your tabular and time series data
Ikigai’s patented Large Graphical Model (LGM) is designed for tabular, time series data. It captures relationships across many dimensions, making it ideal for forecasting and modeling data of all kinds, and especially effective for tackling highly complex multivariate scenarios like inventory planning, pricing strategies, and product placement. Unlike LLMs, the Large Graphical Model does not require vast amounts of historical data. In fact, it can deliver precise results even when analyzing small, sparse data sets - a common issue when new products, suppliers, and distribution centers emerge.
Merchandizing magic with Ikigai
Leveraging the Ikigai platform and its foundational LGM models, aiMatch for data reconciliation, aiCast for forecasting, and aiPlan for scenario-based planning, retailers can finally capitalize on the value of their vast data ecosystem.
With highly accurate and richly informed demand forecasts, retailers can better optimize their inventory levels, placing the right products in the right places at the right time. They can simulate various scenarios to test the effectiveness of different merchandizing strategies, all while gaining insights into the business impact of those strategies across key drivers such as cost, inventory, labor, and suppliers.
See how Ikigai is helping retailers tackle their demand forecasting and merchandizing challenges with its innovative AI platform for tabular and time series data.