Navigating Supply and Demand Harmony with AI: Insights from the Institute of Business Forecasting & Planning (IBF) Townhall
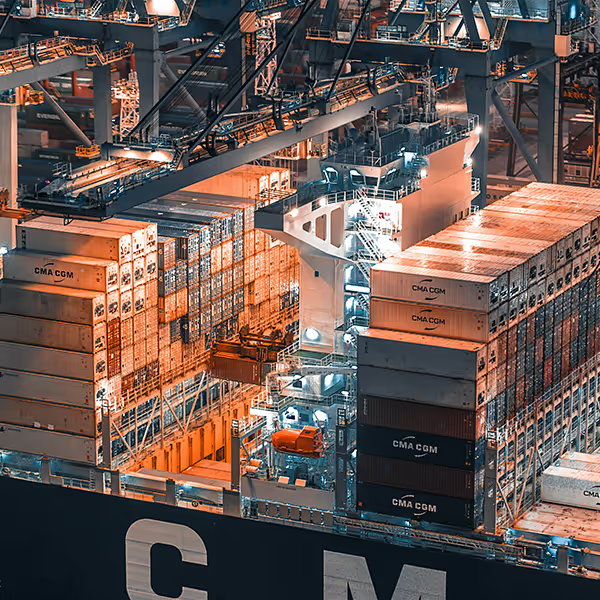
In a volatile world, supply chain managers are turning to AI with the hopes of improved supply chain planning predictability. As AI innovation accelerates, so does the hope of being able to answer the questions that keep us up at night:
- How do I improve my service levels?
- How and where should I expand?
- What products to bring to which market next?
- What transportation route allows me to maximize profits while reducing my carbon footprint?
The ways AI can help supply chain professionals more accurately predict future outcomes, and mitigate evolving risks, was the subject of discussion at the February 28th Institute of Business Forecasting & Planning Virtual Town Hall.
With a full panel including:
- Gerald Verno, Director of Supply Chain Planning & Analytics at Washington Metropolitan Area Transit Authority
- Thiru Viswanathan, Data Scientist Innovation & Portfolio Analytics at Mars Wrigley
- Marianna Vydrevich, Manager of Operations Research & Network Optimization
- Devavrat Shah, Cofounder & CEO Ikigai Labs
A wide variety of topics were discussed, including: AI use cases for supply and demand planners, common data challenges on the road to AI adoption, and new approaches to AI such as Generative AI for time series and tabular data.
But first, the topic on everyones’ mind, will AI take my job?
According to Marianna Vydrevich, AI isn’t ready to take over jobs, but it might be ready for an internship. Or potentially a co-pilot position. According to Thiru Viswanathan, “I see AI as a big efficiency enabler for all the roles, instead of it being a threat to any particular role.”
I see AI as a big efficiency enabler for all the roles, instead of it being a threat to any particular role.
The panelists generally agreed with this sentiment, and as an efficiency driver, shared some of the currently applied use cases shared by the panelists.
AI Use Cases for Supply Chain Productivity
Automating Fuzzy Matching for Data Standardization
Imagine a scenario where a supply chain manager is tasked with analyzing a massive spreadsheet containing thousands of vendor names, broker names, product names, and city names, which are riddled with inconsistencies (such as misspellings and variations like "Mr." versus "Mister.”) To even begin conducting analysis, every version of every input needs to be standardized, and the information must be reviewed for accuracy and completeness. While this is normally an incredibly time consuming and manual task, AI can be used to reduce and streamline the work. By employing advanced fuzzy matching algorithms, AI can automatically identify and standardize variations in the dataset. It can detect similarities between different spellings, account for abbreviations, and recognize variations in naming conventions, resulting in a more complete and usable dataset—including every version of Vendor Name spelled the same (versus Ven. Name, Vender name, and Vendorname.)
Streamlining Decision-Making with Language Models
Picture this: a supply chain team utilizing LLM technology integrated into collaboration platforms like Microsoft Teams. With LLMs at their disposal, team members can pose queries in natural language, cutting through the complexities of traditional data retrieval methods. For instance, they might inquire about the elasticity of a specific product or seek insights into forecasted demand. The LLM swiftly interprets these inquiries, scanning through vast repositories of data and dashboards to provide accurate and timely responses. What once required weeks of data analysis now yields results in mere minutes, empowering supply chain professionals to make informed decisions with unprecedented speed and confidence.
From Supply Chain Efficiency Gains to True Problem Solving
While many of today’s AI use cases are efficiency driven, as AI continues to mature, the application focus is shifting towards more profound problem-solving capabilities, such as better prediction and management of supply and demand. As Marianne Vydrevich put it, "Computational power is increasing, so models can get more granular, and you can build in more business constraints, like service level." With AI, supply chain professionals can conduct sophisticated simulations, analyzing various demand scenarios and their impact on key performance indicators such as stockouts.
Gerald Verno echoed this sentiment, emphasizing the importance of understanding trade-offs in supply chain management. He highlighted the challenges faced in his role at the Washington Metropolitan Area Transit Authority, predicting ridership post-pandemic and the need to adapt to unforeseen disruptions, such as events like concerts that can significantly impact operational plans. “Today, everything's fine, tomorrow, Taylor Swift announces a concert downtown. That's a major impact to our system that then we have to then retool everything, and it happens a lot.”
Today, everything's fine, tomorrow, Taylor Swift announces a concert downtown. That's a major impact to our system that then we have to then retool everything, and it happens a lot.
Historically, it has been challenging for supply chain teams to walk the tightrope of balancing supply with demand, especially because there are things that can't be controlled, like uncertainty in the market. That's where accurate prediction and forecasting become crucial. In order to effectively balance supply and demand, organizations require access to accurate and comprehensive data. This data encompasses various sources, including first-party data related to demand forecasting and third-party data concerning product information, inventory status, and more. In many cases, this data is sparse, incomplete, inaccurate, or spread across many systems.
How Generative AI for Time Series Data Will Finally Solve the Business Intelligence Gap
According to panelist Devavrat Shah, the biggest benefit AI organizations like Ikigai bring to supply chain managers is the ability to finally capture, clean, and reconcile data in one place to make sense of it in a way that enables true insight. “In all enterprises, data primarily revolves around people, products, finances, sales, and the complex interplay between them,” shared Shah. “Every supply chain manager knows the challenge of making BI work when there is bad data, but large graphical models are helping companies leverage their data for better insights by building probabilistic views of data from different sources, facilitating insights even from imperfect datasets.”
Large graphical models are helping companies leverage their data for better insights by building probabilistic views of data from different sources, facilitating insights even from imperfect datasets.
Generative AI solutions built on graphical models aim to create a probabilistic understanding of data from diverse sources. Even if the data tables differ in column names or content, graphical models identify relationships and potential matches across datasets, and employ fuzzy matching techniques to account for spelling errors and other discrepancies, aiming to reconcile and combine data effectively. With generative AI, even data that has missing information and gaps can be leveraged to create extremely accurate predictions and model a wide variety of planning scenarios.
With Ikigai, Shah built in methods of explainability, as there's always a level of uncertainty in probabilistic matching. That’s why Ikigai leverages Expert in the Loop (XitL) technology, so subject matter experts within the organization can quickly refine the matching process and increase the system’s confidence levels. Finally, because Ikigai can bring in time-series, tabular data from any source, it allows supply chain managers to leverage industry-wide data rather than relying solely on individual company data, which gives teams the ability to forecast based on comprehensive industry information, including weather, stock market, or social media trends, which can provide early signals of demand shifts.
In conclusion, the journey of AI in supply chain management is evolving towards true problem-solving. By addressing challenges related to data quality, accessibility, and integration, supply chain professionals can unlock the full potential of AI to drive innovation and resilience in an increasingly dynamic business environment.